Electrocardiosignal automatic analysis method based on deep learning
A deep learning and electrocardiographic signal technology, applied in neural learning methods, instruments, biological neural network models, etc., can solve the problems of limited nonlinear fitting ability, reduced accuracy of classification algorithms, and prone to misclassification, etc., and achieve high-efficiency waveforms. The effect of feature extraction, good classification results, good compatibility
- Summary
- Abstract
- Description
- Claims
- Application Information
AI Technical Summary
Problems solved by technology
Method used
Image
Examples
Embodiment Construction
[0027] The present invention will be described in detail below in conjunction with the accompanying drawings.
[0028] The present invention uses a deep learning model to process 12-lead ECG data, uses a one-dimensional convolutional neural network as a basis, and uses a LAD network architecture to train the deep learning model. The deep learning model is used as a black box, and there is no need to manually specify the use of certain ECG signal features for classification, and the computer can learn the classification process by itself. At the same time, the input of the deep learning model is all the ECG signal data (rather than a certain feature of the ECG signal), which reduces the loss of information.
[0029] The present invention uses a one-dimensional convolutional neural network (CNN) to directly process the original electrocardiographic data, which can eliminate the step of signal preprocessing on the electrocardiographic data, that is, remove noise such as baseline ...
PUM
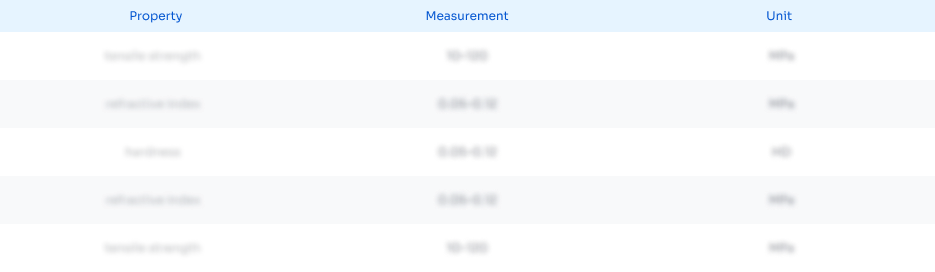
Abstract
Description
Claims
Application Information
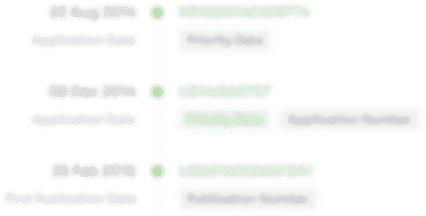
- R&D Engineer
- R&D Manager
- IP Professional
- Industry Leading Data Capabilities
- Powerful AI technology
- Patent DNA Extraction
Browse by: Latest US Patents, China's latest patents, Technical Efficacy Thesaurus, Application Domain, Technology Topic, Popular Technical Reports.
© 2024 PatSnap. All rights reserved.Legal|Privacy policy|Modern Slavery Act Transparency Statement|Sitemap|About US| Contact US: help@patsnap.com