Deep learning non-neighborhood equalization processing method and system for water color remote sensing image
A remote sensing image and deep learning technology, applied in the field of image processing, can solve the problems of increasing background and noise contrast, lack of intelligence, image gray value reduction, etc., to achieve high definition, strong adaptability and robustness, and improve The effect of image signal-to-noise ratio
- Summary
- Abstract
- Description
- Claims
- Application Information
AI Technical Summary
Problems solved by technology
Method used
Image
Examples
Embodiment 1
[0055] figure 1 This is a flowchart of a deep learning non-neighborhood averaging processing method for water color remote sensing images provided by the embodiments of this application, such as figure 1 As shown, a deep learning non-neighboring averaging processing method for water color remote sensing images provided by the present invention includes:
[0056] Step S101, collecting an original image to be processed, the original image being a noisy satellite water color image;
[0057] Step S102, input the original image to be processed into the non-local mean model of the preset deep convolutional neural network framework;
[0058] Step S103, using the convolution kernel of the first layer of the N convolutional layers of the non-local mean model of the preset deep convolutional neural network framework to perform convolution processing on the original image to obtain the original image characteristics of the original image, where N is A positive integer greater than 17;
[0059] S...
Embodiment 2
[0089] image 3 This is a flowchart of another method for deep learning non-neighborhood averaging processing of water color remote sensing images provided by the embodiments of this application, such as image 3 As shown, another method for deep learning non-neighbor averaging processing of water color remote sensing images provided by the present invention includes:
[0090] Step S201: Input the noisy satellite water color image into the non-local mean model of the deep convolutional neural network framework that has been trained.
[0091] Step S202: Input 64 image feature maps with a size of 35×35 to the first layer of the non-local mean model of the deep convolutional neural network framework, and process them through 64 3×3×3 convolution kernels in the first layer , Output 64 feature maps with a size of 35×35, that is, 35×35×64 feature images.
[0092] In this step, the first layer of the non-local mean model of the deep convolutional neural network framework is the feature extr...
Embodiment 3
[0109] Figure 4 This is a schematic structural diagram of a deep learning non-neighborhood averaging processing system for water color remote sensing images provided by the embodiments of this application, such as Figure 4 As shown, the present invention also provides a deep learning non-neighborhood averaging processing system for water color remote sensing images, including:
[0110] The acquisition module 301 is used to acquire the original image to be processed, and the original image is a noisy satellite water color image;
[0111] The calculation module 302 is used to input the original image to be processed into the non-local mean model of the preset deep convolutional neural network framework;
[0112] The first processing module 303 is used to perform convolution processing on the original image by using the convolution kernel of the first layer of the N convolutional layers of the non-local mean model of the non-local mean model of the preset deep convolutional neural ne...
PUM
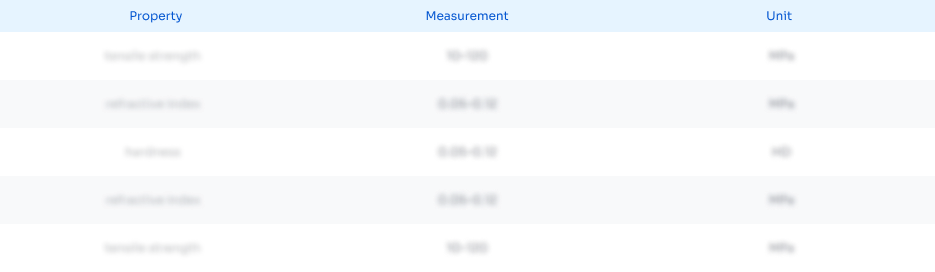
Abstract
Description
Claims
Application Information
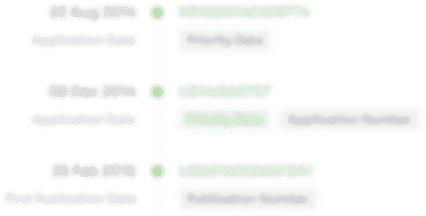
- R&D Engineer
- R&D Manager
- IP Professional
- Industry Leading Data Capabilities
- Powerful AI technology
- Patent DNA Extraction
Browse by: Latest US Patents, China's latest patents, Technical Efficacy Thesaurus, Application Domain, Technology Topic, Popular Technical Reports.
© 2024 PatSnap. All rights reserved.Legal|Privacy policy|Modern Slavery Act Transparency Statement|Sitemap|About US| Contact US: help@patsnap.com