Precise elimination method for laser point cloud noise and redundant data
A technology of laser point cloud data and redundant data, which is applied in image data processing, character and pattern recognition, instruments, etc., can solve the problems of low automation, smooth results, and low efficiency, and achieve high automation and reduce Hollow situation, the effect of improving the denoising speed
- Summary
- Abstract
- Description
- Claims
- Application Information
AI Technical Summary
Problems solved by technology
Method used
Image
Examples
Embodiment Construction
[0080] The technical solution of the method for accurately eliminating noise and redundant data of laser point cloud provided by the present invention is further described below with reference to the accompanying drawings, so that those skilled in the art can better understand the present invention and implement it.
[0081] There are errors in the laser scanning process, which makes the laser point cloud data contain noise, and the noise must be removed from the scanned laser point cloud data; the laser point cloud data has the characteristics of a large amount of data, and the redundancy in the laser point cloud data must be eliminated. data. In view of this, the present invention starts from the aspects of k-nearest neighborhood and bilateral filtering laser point cloud data fusion denoising, boundary-preserving surface variational adaptive simplification method, etc., to ensure the accuracy and reliability of laser point cloud data after preprocessing.
[0082] The present...
PUM
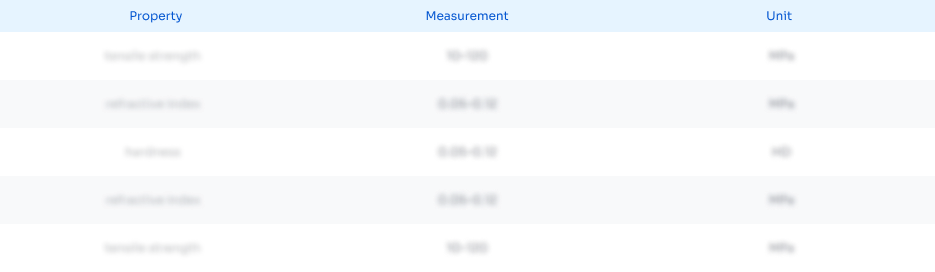
Abstract
Description
Claims
Application Information
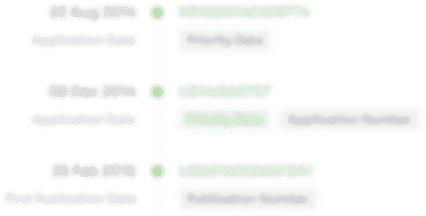
- R&D Engineer
- R&D Manager
- IP Professional
- Industry Leading Data Capabilities
- Powerful AI technology
- Patent DNA Extraction
Browse by: Latest US Patents, China's latest patents, Technical Efficacy Thesaurus, Application Domain, Technology Topic.
© 2024 PatSnap. All rights reserved.Legal|Privacy policy|Modern Slavery Act Transparency Statement|Sitemap