Common colon disease classification method based on deep neural network and auxiliary system
A deep neural network and disease classification technology, applied in the field of common colon disease classification methods and auxiliary systems, can solve the problems of large subjective influencing factors, time-consuming and labor-intensive colonoscopy, high missed detection rate and false detection rate. Improve diagnostic efficiency, improve accuracy, reduce missed detection rate and false detection rate
- Summary
- Abstract
- Description
- Claims
- Application Information
AI Technical Summary
Problems solved by technology
Method used
Image
Examples
Embodiment 1
[0042] This embodiment is a method for classifying common colon diseases based on a deep neural network, the flow chart of which is shown in figure 1 , wherein the method includes the following steps:
[0043] S1. Obtain the colonoscopy image data, and mark the name of the lesion contained in the image for the data sample. If there is no lesion in the image, mark it as normal; the lesion types included in this example include cancer, polyp and inflammation. If there is no lesion in the image , it is marked as normal.
[0044]S2. Divide the data samples of the same label into a data set, and divide each data set into a training set, a verification set and a test set, wherein the training set and the verification set are used for model training and saving model parameters respectively, and the test set is used for To verify the final model effect; when training a neural network, too small a training set sample size will cause the network to overfit and affect the training effec...
Embodiment 2
[0058] In this embodiment, on the basis of Embodiment 1, an auxiliary system for common colon diseases based on a deep neural network is proposed. This system is divided into two main modules: a model training module and an auxiliary diagnosis module. The model training modules include:
[0059] (1) The data acquisition module is used to acquire colonoscopy image data, and label the names of the lesions contained in the data sample images; in this example, the images without lesions are marked as normal, and the images with lesions include cancer, polyps , three types of inflammation.
[0060] (2) The model training module is used to train the corresponding colonoscopy image classification model based on data sets of different classification tasks; A lesion type classification model is trained on datasets of , polyps, and inflammation.
[0061] (3) Model identification module, used to input the corresponding classification model to the colonoscope image to be classified to o...
PUM
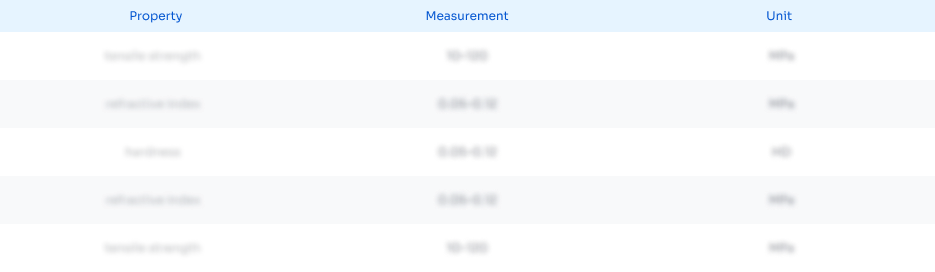
Abstract
Description
Claims
Application Information
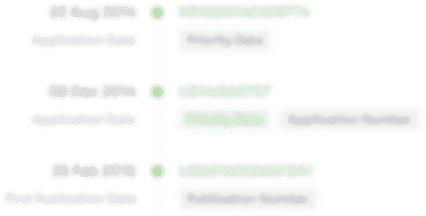
- R&D Engineer
- R&D Manager
- IP Professional
- Industry Leading Data Capabilities
- Powerful AI technology
- Patent DNA Extraction
Browse by: Latest US Patents, China's latest patents, Technical Efficacy Thesaurus, Application Domain, Technology Topic, Popular Technical Reports.
© 2024 PatSnap. All rights reserved.Legal|Privacy policy|Modern Slavery Act Transparency Statement|Sitemap|About US| Contact US: help@patsnap.com