Image motion blur removing method based on improved cyclic generative adversarial network
A motion blur, improved technology, applied in biological neural network models, image enhancement, image analysis, etc., can solve the problems of lack of feature consistency, inaccurate blur kernel estimation, and reduced image recognition accuracy.
- Summary
- Abstract
- Description
- Claims
- Application Information
AI Technical Summary
Problems solved by technology
Method used
Image
Examples
Embodiment Construction
[0064] The present invention will be further described below in conjunction with the accompanying drawings and embodiments.
[0065] figure 1 It is a flowchart of a method for removing image motion blur based on an improved recurrent generation confrontation network in an example of the present invention, including the following steps:
[0066] Step 1. Select an existing public blur-clear image dataset, and construct an unpaired blur-clear dataset as a training dataset and a test dataset. In this embodiment, the published GOPRO_Large dataset is used, and the GOPRO_Large dataset includes street view pictures taken by a high-frame camera and artificially synthesized blurred pictures.
[0067] To further improve the generalization ability and recognition accuracy of the model for motion blur including non-vertical angles of handheld objects, the network model is trained using data enhancement methods, and the input training samples are randomly adjusted for angles, expressed as:...
PUM
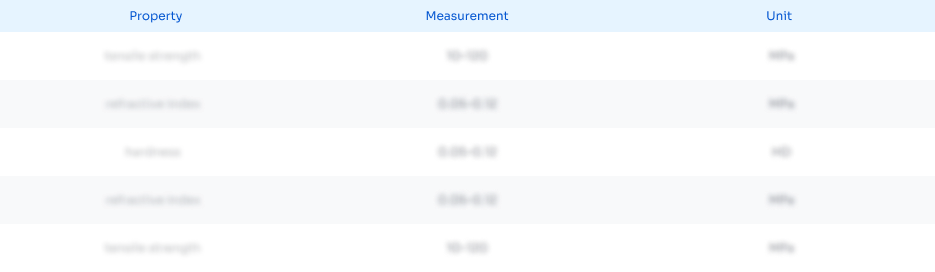
Abstract
Description
Claims
Application Information
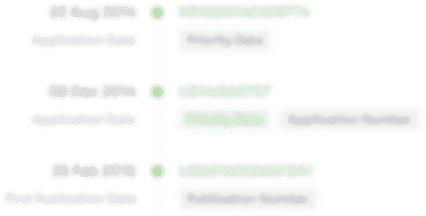
- R&D
- Intellectual Property
- Life Sciences
- Materials
- Tech Scout
- Unparalleled Data Quality
- Higher Quality Content
- 60% Fewer Hallucinations
Browse by: Latest US Patents, China's latest patents, Technical Efficacy Thesaurus, Application Domain, Technology Topic, Popular Technical Reports.
© 2025 PatSnap. All rights reserved.Legal|Privacy policy|Modern Slavery Act Transparency Statement|Sitemap|About US| Contact US: help@patsnap.com