Nuclear staining cell counting method based on deep learning of incomplete marker, computer equipment and storage medium
A deep learning and cell counting technology, applied in the field of deep learning, can solve the problems of single color cells, difficult to complete intelligent learning, low accuracy, etc.
- Summary
- Abstract
- Description
- Claims
- Application Information
AI Technical Summary
Problems solved by technology
Method used
Image
Examples
Embodiment 1
[0094] A method for counting nuclear stained cells based on deep learning of incomplete marking, comprising the following steps:
[0095] (1) Create annotation data
[0096] Aiming at the current problems of cell counting, the present invention is based on the artificial intelligence cell counting scheme based on deep learning, combined with the characteristics of counting, and proposes an efficient, robust and easy-to-apply cell labeling method.
[0097] Load the pathological image to the labeling software to obtain all sub-images and sub-mask image data pairs of positive cells;
[0098] (2) Training model
[0099] Obtain all subimages and the submask image data pair of positive cells by step (1) to be the positive cell training set; Obtain all subimages and the submask image data pair of negative cells by step (1) to be negative cell training set;
[0100] Train the convolutional neural network model of the same structure through the positive cell training set and the neg...
Embodiment 2
[0107] According to a kind of method for counting nuclear stained cells based on the deep learning of incomplete labeling described in Example 1, the difference is that:
[0108] In step (1), make label data, such as image 3 shown, including the following steps:
[0109] a. Load the pathological image to the labeling software; usually it is not necessary to label the whole pathological slice image, just take an image with a size of about 700 in a certain field of view.
[0110] b. Establish a positive cell label, and click on the individual center points of all positive cells in the pathological image, and use the individual center points of each positive cell as the center of the rectangle to mark a rectangular block to form a real mask image of positive cells;
[0111] c. Establish a negative cell label, and click on the individual center points of all negative cells in the pathological image, and use the individual center points of each negative cell as the center of the ...
Embodiment 3
[0131] According to a kind of method for counting nuclear stained cells based on the deep learning of incomplete labeling described in embodiment 2, the difference is:
[0132] like figure 2 As shown, the inference phase includes the following steps:
[0133] The DCP-Net provided by the present invention can input an image of any size, but when the input image is too large, one-time input is likely to cause insufficient computing resources. Input the complete cell image into the network for automatic and intelligent cell identification, or input identification and detection one by one in a block-by-block manner when computing resources are insufficient.
[0134] A. Divide the pathological image to be detected into several sub-images of equal size;
[0135] B. Carry out mirror filling to the lower border and the right border of the sub-image obtained after the processing in step A, so that the size of the sub-image after mirror filling is a multiple of the preset sub-image s...
PUM
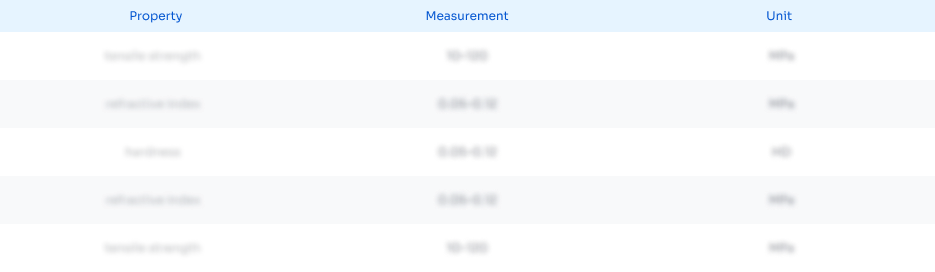
Abstract
Description
Claims
Application Information
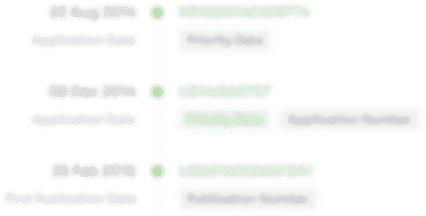
- R&D Engineer
- R&D Manager
- IP Professional
- Industry Leading Data Capabilities
- Powerful AI technology
- Patent DNA Extraction
Browse by: Latest US Patents, China's latest patents, Technical Efficacy Thesaurus, Application Domain, Technology Topic, Popular Technical Reports.
© 2024 PatSnap. All rights reserved.Legal|Privacy policy|Modern Slavery Act Transparency Statement|Sitemap|About US| Contact US: help@patsnap.com