Machine learning-based bimodal image omics ground glass nodule classification method
A radiomics and machine learning technology, applied in the medical field, can solve the problem of not considering nonlinear factors, and achieve the effect of improving prediction efficiency, good robustness and high accuracy
- Summary
- Abstract
- Description
- Claims
- Application Information
AI Technical Summary
Problems solved by technology
Method used
Image
Examples
Embodiment
[0024] A machine learning-based dual-modality radiomics classification method for ground-glass nodules, such as Figure 1-2 shown, including the following steps:
[0025] Step 1: Case data collection
[0026] Collection accepted for suspicious pulmonary GGN 18 Patients with F-FDG PET / CT examination;
[0027] Inclusion criteria: 0.8cm≤GGN≤3cm; PET / CT scan and breath-hold chest CT scan were performed at the same time; the lesion was surgically resected within 1 month after the PET / CT examination and the pathological data were complete;
[0028] Exclusion criteria: patients with poor image quality or lesions below 64 voxels in PET imaging; patients who have received any anti-tumor therapy within 5 years; patients with lung cancer stage IB and above; patients with fasting blood glucose > 11.1mmol / L ; Patients with severely impaired liver function (serum alanine aminotransferase or aspartate aminotransferase exceeds 5 times the upper limit of normal value);
[0029] Step 2: Ima...
PUM
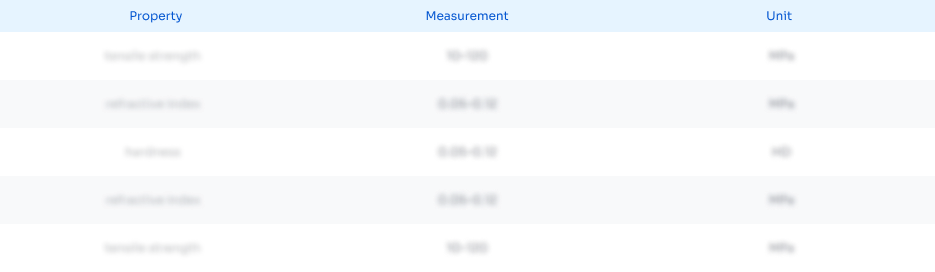
Abstract
Description
Claims
Application Information
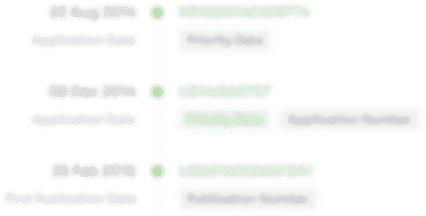
- R&D
- Intellectual Property
- Life Sciences
- Materials
- Tech Scout
- Unparalleled Data Quality
- Higher Quality Content
- 60% Fewer Hallucinations
Browse by: Latest US Patents, China's latest patents, Technical Efficacy Thesaurus, Application Domain, Technology Topic, Popular Technical Reports.
© 2025 PatSnap. All rights reserved.Legal|Privacy policy|Modern Slavery Act Transparency Statement|Sitemap|About US| Contact US: help@patsnap.com