Binocular inertial simultaneous localization and mapping method based on point-line feature fusion
A technology of feature fusion and map construction, which is applied in image enhancement, image analysis, 3D modeling, etc. It can solve the problem that adjacent similar line segments and long line segments are easily divided into multiple short line segments, multiple outliers, line segment repetition detection, etc. problem, to achieve the effect of optimizing the extraction quality is not high, high-precision pose estimation, and reducing the false matching rate
- Summary
- Abstract
- Description
- Claims
- Application Information
AI Technical Summary
Problems solved by technology
Method used
Image
Examples
Embodiment 1
[0155] The simulation experiment is carried out using public datasets, where the datasets are the most difficult subsequences MH_04_difficult, MH_05_difficult, V1_03_difficult, V2_03_difficult to extract from the four scenes in the EuRoC dataset [31].
[0156] In step S1, the point feature and line feature are detected and tracked. In the point feature detection and track, the corner points are extracted as feature points, and the KLT optical flow method is used to realize the feature point tracking, and the large difference is eliminated based on the reverse optical flow tracking. point.
[0157] In line feature detection and tracking, line features are extracted through the following steps:
[0158] S101, image grayscale: For the color image input by the sensor, the weighted average is converted into a grayscale image according to the sampling values of each channel of the image;
[0159]S102. Noise reduction: use a Gaussian filter to filter out noise and smooth the image...
experiment example 1
[0221] The time-consuming and line feature extraction quantity of each frame in Example 1, Comparative Example 1, and Comparative Example 2 were counted, and the results are shown in Table 1.
[0222] Table I
[0223]
[0224] The machine hall extraction effect under the EuRoC data set is as follows Figure 4~6 shown, where Figure 4 Extract the effect for Comparative Example 1, Figure 5 Extract the effect for Comparative Example 2, Image 6 Extract effect for embodiment 1, from table one and Figure 4~6 It can be seen that the number of line segments extracted in Comparative Example 1 is the largest, followed by Comparative Example 2, and the least in Example 1. There are a large number of short line segment features in Comparative Example 1 and Comparative Example 2, which increases the calculation cost of line segment detection and matching, and some The division of long line segments into many short line segments and the existence of adjacent line segments complica...
experiment example 2
[0227] Statistical embodiment 1, comparative example 3, the motion estimation error in comparative example 4, adopt the accuracy of absolute track error evaluation algorithm, promptly calculate the root mean square error (root mean square error) of the Euclidean distance between estimated pose and true pose , RMSE) and the maximum value. When comparing with the real trajectory, the EVO (evaluation of odometry and SLAM) tool is used for data alignment and error calculation, where the root mean square error is the final error obtained by considering both translation and rotation.
[0228] The results are shown in Table II.
[0229] Table II
[0230]
[0231] It can be seen from Table 2 that due to the high quality of line feature extraction in Example 1, the positioning accuracy is better than that of Comparative File 3 and Comparative File 4. Except for the two simple sequences of MH_01_easy and MH_02_easy, the accuracy of the two simple sequences is slightly lower than tha...
PUM
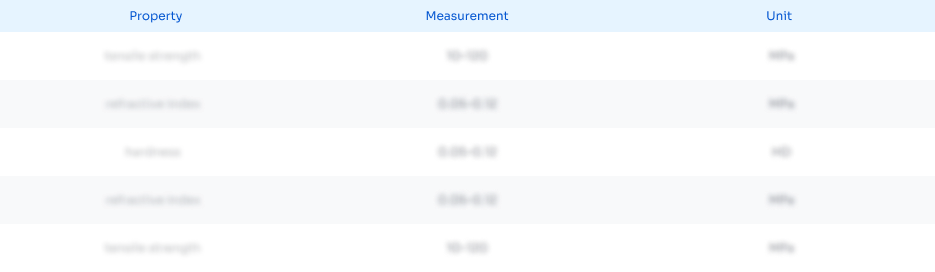
Abstract
Description
Claims
Application Information
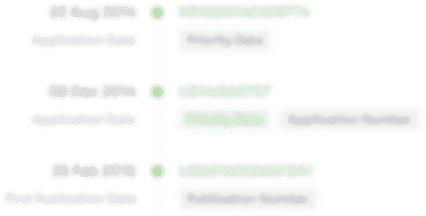
- R&D
- Intellectual Property
- Life Sciences
- Materials
- Tech Scout
- Unparalleled Data Quality
- Higher Quality Content
- 60% Fewer Hallucinations
Browse by: Latest US Patents, China's latest patents, Technical Efficacy Thesaurus, Application Domain, Technology Topic, Popular Technical Reports.
© 2025 PatSnap. All rights reserved.Legal|Privacy policy|Modern Slavery Act Transparency Statement|Sitemap|About US| Contact US: help@patsnap.com