Small sample SAR image target recognition method based on improved prototype network
A prototype network, target recognition technology, applied in the field of SAR automatic target recognition, can solve hundreds of problems, and achieve the effect of reducing sensitivity, improving recognition accuracy, and improving recognition performance
- Summary
- Abstract
- Description
- Claims
- Application Information
AI Technical Summary
Problems solved by technology
Method used
Image
Examples
Embodiment 1
[0034] refer to figure 1 , a kind of small sample SAR image object recognition method based on improved prototype network provided by the present invention, comprises the following steps:
[0035] Step 1. Obtain the training sample set and the small sample SAR image set to be identified; select C×m SAR images by category from the training sample set as the training support set, and the remaining A SAR image to form a training query set; similarly, the small-sample SAR image set to be identified is divided into a labeled support set and a query set to be identified;
[0036] Among them, the target category in the small sample SAR image set to be identified is different from the target category in the training sample set; the number of target categories in the two is the same, C is the total number of target categories to be trained, and m is the SAR of each target category in the training support set number of images, is the total number of SAR images corresponding to the k...
Embodiment 2
[0070] refer to figure 2 , a deep convolution-bidirectional long-short-time prototype neural network, including sequentially connected deep convolution-bidirectional long-short-time neural network, a prototype module and a classifier based on Euclidean distance; the deep convolution-bidirectional long-short-time The neural network includes a deep convolutional neural network and a bidirectional long-short-time neural network; the deep convolutional neural network includes a plurality of convolutional modules connected in sequence and a fully connected layer; each convolutional module includes a convolutional layer connected in sequence, a ReLU Activation layers, batch normalization layers, and max pooling layers.
[0071] In this embodiment, a maximum pooling layer may also be set after multiple convolutional layers, ReLU activation layers, and batch normalization layers.
[0072] like figure 2Shown: In this example, the construction includes eight convolutional layers, ei...
Embodiment 3
[0075] refer to image 3 , a deep convolution-bidirectional long-short-time prototype neural network training method, comprising the following steps:
[0076] Step 1. Obtain training sample set and test sample set; select C×m SAR images by category from the training sample set as the training support set, and the remaining SAR images form a training query set; similarly, the test sample set is divided into a test support set and a test query set;
[0077] Among them, C is the total number of target categories to be trained, m is the number of SAR images of each target category in the training support set, is the total number of SAR images corresponding to the kth class target in the training sample set; the test support set is a labeled sample, and the test query set is an unlabeled sample;
[0078] Step 2, using the training support set and the training query set to train the deep convolution-bidirectional long-short-time prototype neural network to obtain the trained dee...
PUM
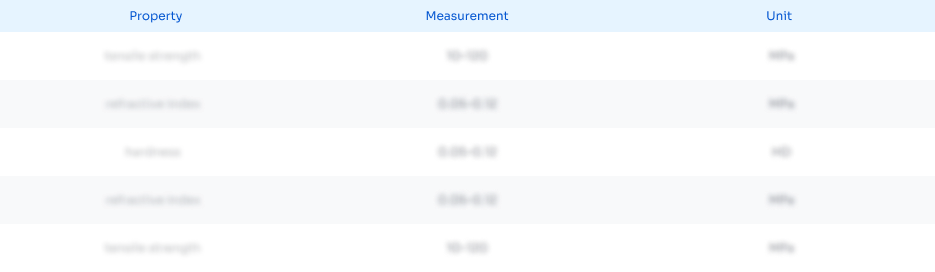
Abstract
Description
Claims
Application Information
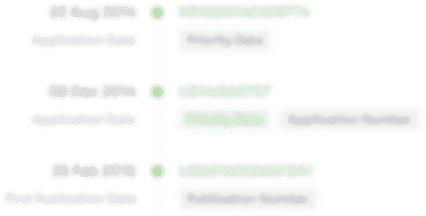
- R&D Engineer
- R&D Manager
- IP Professional
- Industry Leading Data Capabilities
- Powerful AI technology
- Patent DNA Extraction
Browse by: Latest US Patents, China's latest patents, Technical Efficacy Thesaurus, Application Domain, Technology Topic, Popular Technical Reports.
© 2024 PatSnap. All rights reserved.Legal|Privacy policy|Modern Slavery Act Transparency Statement|Sitemap|About US| Contact US: help@patsnap.com