Machine learning-based rapid forecasting method for dynamic response of hull grillage structure under underwater explosion load
A technology of underwater explosion and machine learning, which is applied in the field of ship damage under underwater explosion load, can solve the problems of difficult models and high test costs, and achieve the effects of improved accuracy, rapid prediction, and saving calculation time
- Summary
- Abstract
- Description
- Claims
- Application Information
AI Technical Summary
Problems solved by technology
Method used
Image
Examples
specific Embodiment 1
[0050] according to Figure 1 to Figure 5 As shown, the present invention provides a method for fast prediction of the dynamic response of the hull grillage structure under the underwater explosion load based on machine learning, comprising the following steps:
[0051] A machine learning-based rapid prediction method for the dynamic response of hull grille structures under underwater explosion loads, comprising the following steps:
[0052] Step 1: Use any Euler-Lagrangian method to numerically calculate the response of the hull grille structure under underwater explosion loads to obtain data samples;
[0053] The step 1 is specifically:
[0054] Step 1.1: Determine the size of the water area according to the radius of the largest bubble produced by the underwater explosion of a certain equivalent explosive. The size of the water area should be 2 to 4 times the maximum diameter of the bubble produced by a certain equivalent explosive at the corresponding water depth;
[005...
specific Embodiment 2
[0087] The size of the water area in step 1 should be 2 to 4 times the maximum diameter of the air bubbles produced by the cartridge at the corresponding water depth, and the maximum diameter of the air bubbles is calculated using the Geers-Hunter model:
[0088]
[0089] In the formula: m c is the quality of the drug pack, a c is the radius of drug inclusion, ρ f is fluid density, K, k, A, B are explosive material parameters.
[0090] By time-integrating equation (1), the volume change rate and the volume change law with time can be obtained respectively:
[0091]
[0092]
[0093] Then solve the bubble radius a and the vertical migration u of the bubble produced by the underwater explosion:
[0094]
[0095]
[0096]
[0097]
[0098]
[0099] In the formula: g is the acceleration due to gravity, K c and r are explosive material parameters, C D is the fluid resistance coefficient, ρ c is the explosive density, p I is the hydrostatic press...
specific Embodiment 3
[0133] Determine the size of the water area according to the radius of the largest bubble produced by the underwater explosion of a certain equivalent explosive. The size of the water area should be 2 to 4 times the maximum diameter of the bubble produced by a certain equivalent explosive at the corresponding water depth; grid size, and the S-ALE method is used to discretize the water area and air, so that the grid size within the maximum diameter range of the bubble is smaller, and the grid size in other areas is twice the grid size within the maximum diameter range; then, the hull plate The frame structure is modeled and the structure is consistent with the minimum grid size of the water area to complete the model establishment. Such as figure 2 with image 3 As shown, the arbitrary Euler-Lagrangian method (ALE) is used to calculate the dynamic response of the hull grille structure under the underwater explosion load, and the coordinates of each node of the structure and t...
PUM
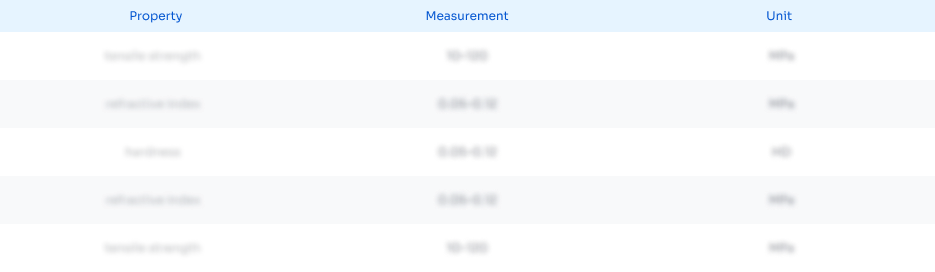
Abstract
Description
Claims
Application Information
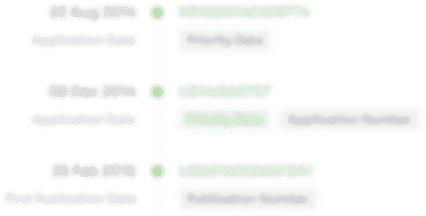
- R&D Engineer
- R&D Manager
- IP Professional
- Industry Leading Data Capabilities
- Powerful AI technology
- Patent DNA Extraction
Browse by: Latest US Patents, China's latest patents, Technical Efficacy Thesaurus, Application Domain, Technology Topic.
© 2024 PatSnap. All rights reserved.Legal|Privacy policy|Modern Slavery Act Transparency Statement|Sitemap