Deep learning remote sensing image semantic segmentation method and system based on U-NET
A remote sensing image and semantic segmentation technology, which is applied in neural learning methods, instruments, biological neural network models, etc., can solve the problem of large workload, long time-consuming development of high-resolution remote sensing satellites, and difficulties in meeting the requirements of segmentation accuracy and segmentation target categories And other issues
- Summary
- Abstract
- Description
- Claims
- Application Information
AI Technical Summary
Problems solved by technology
Method used
Image
Examples
Embodiment 1
[0079] see figure 1 , figure 1 It is a schematic flowchart of a U-NET-based deep learning remote sensing image semantic segmentation method disclosed in an embodiment of the present invention. Such as figure 1 As shown, the U-NET-based deep learning remote sensing image semantic segmentation method may include the following steps.
[0080] 101. Perform correction and super-resolution reconstruction on the initial remote sensing data to obtain reconstructed remote sensing data.
[0081] In this embodiment, the low-resolution initial remote sensing data is corrected and reconstructed to provide standardized high-resolution remote sensing data for subsequent segmentation.
[0082] As an optional implementation, atmospheric correction and radiation correction are performed on the initial remote sensing data to obtain the corrected image; denoising and upsampling are performed on the corrected image to obtain the characteristic image; the nonlinear features contained in the char...
Embodiment 2
[0099] see figure 2 , figure 2 It is a schematic structural diagram of a U-NET-based deep learning remote sensing image semantic segmentation system disclosed in an embodiment of the present invention. Such as figure 2 As shown, the U-NET-based deep learning remote sensing image semantic segmentation system may include the following contents.
[0100] A data reconstruction unit 201, configured to perform correction and super-resolution reconstruction on the initial remote sensing data to obtain reconstructed remote sensing data;
[0101] The sample library construction unit 202 is used to classify and preprocess the reconstructed remote sensing data and construct a remote sensing sample library;
[0102] The model building unit 203 is used to set up a segmented network model based on U-NET, and use the Keras artificial neural network library as a learning framework to predict and classify the remote sensing sample library to obtain a basic training data set;
[0103] An...
Embodiment 3
[0130] see image 3 , image 3 It is a schematic structural diagram of another U-NET-based deep learning remote sensing image semantic segmentation system disclosed in the embodiment of the present invention. Such as image 3 As shown, the U-NET-based deep learning remote sensing image semantic segmentation system can include:
[0131] A memory 301 storing executable program codes;
[0132] a processor 302 coupled to the memory 301;
[0133] Wherein, the processor 302 invokes the executable program code stored in the memory 301 to execute figure 1 A deep learning remote sensing image semantic segmentation method based on U-NET.
[0134] The embodiment of the present invention discloses a computer-readable storage medium, which stores a computer program, wherein the computer program enables the computer to execute figure 1 A deep learning remote sensing image semantic segmentation method based on U-NET.
[0135] The embodiment of the present invention also discloses a comp...
PUM
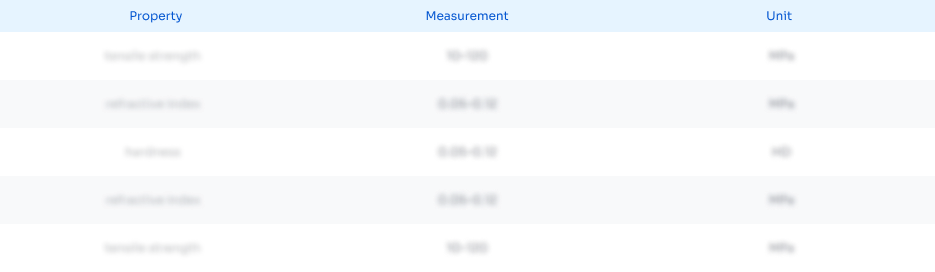
Abstract
Description
Claims
Application Information
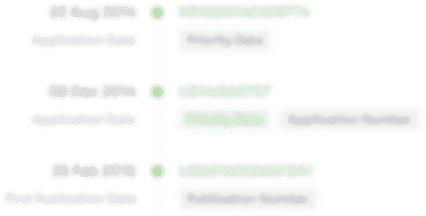
- R&D Engineer
- R&D Manager
- IP Professional
- Industry Leading Data Capabilities
- Powerful AI technology
- Patent DNA Extraction
Browse by: Latest US Patents, China's latest patents, Technical Efficacy Thesaurus, Application Domain, Technology Topic, Popular Technical Reports.
© 2024 PatSnap. All rights reserved.Legal|Privacy policy|Modern Slavery Act Transparency Statement|Sitemap|About US| Contact US: help@patsnap.com