Supercritical fluid heat transfer correlation type proxy model construction method based on machine learning
A supercritical fluid and surrogate model technology is applied in the field of fluid heat transfer correlation surrogate model construction, which can solve the problems of difficult empirical correlation prediction and poor accuracy of heat transfer, and achieve the effect of less time required for calculation and high prediction accuracy.
- Summary
- Abstract
- Description
- Claims
- Application Information
AI Technical Summary
Problems solved by technology
Method used
Image
Examples
specific Embodiment
[0117] Attached below figure 1 - attached Figure 4 , with supercritical CO 2 The fluid example further introduces the technical solution implemented by the present invention.
[0118] according to figure 1 The process of establishing the heat transfer correlation model of the present invention is further described in detail.
[0119] 1. With supercritical CO 2 The data processing of the heat transfer experiment in the working fluid tube is taken as an example, respectively from the existing public documents Kim et al. al. (DOI: doi.org / 10.1016 / j.expthermflusci.2010.06.001, DOI: doi.org / 10.1016 / j.ijheatfluidflow.2010.06.013), Lei et al. (DOI: doi.org / 10.3390 / app7121260 ), Kimet al. (DOI: 10.1016 / j.nucengdes.2010.07.002, DOI: 10.1016 / j.supflu.2011.04.014), Song et al. (DOI: 10.1016 / j.supflu.2007.11.013), Gupta et al.(DOI:doi.org / 10.1115 / ICONE21-16453), H.Zahlan et al.(DOI:doi.org / 10.1016 / j.nucengdes.2015.04.013) collected, organized and established 10552 data points CO ...
PUM
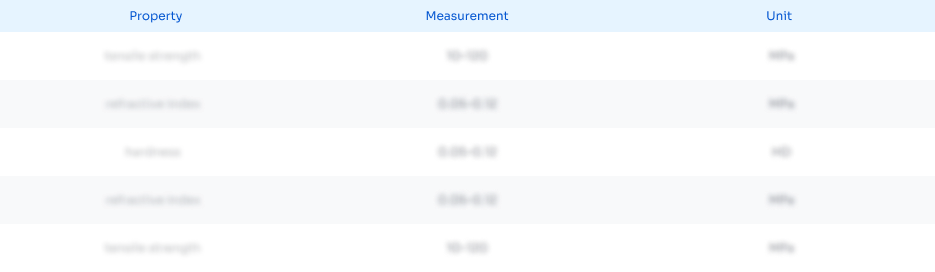
Abstract
Description
Claims
Application Information
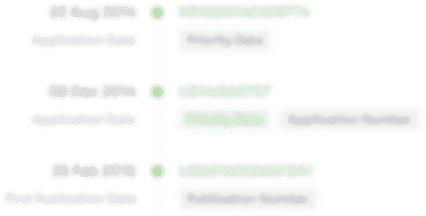
- R&D Engineer
- R&D Manager
- IP Professional
- Industry Leading Data Capabilities
- Powerful AI technology
- Patent DNA Extraction
Browse by: Latest US Patents, China's latest patents, Technical Efficacy Thesaurus, Application Domain, Technology Topic, Popular Technical Reports.
© 2024 PatSnap. All rights reserved.Legal|Privacy policy|Modern Slavery Act Transparency Statement|Sitemap|About US| Contact US: help@patsnap.com