Image generation method based on adaptive gradient cutting under differential privacy protection
A differential privacy, image generation technology, applied in 2D image generation, image enhancement, image analysis and other directions, can solve problems such as unreasonable cropping and adding noise, and achieve the effect of fast training convergence and high image quality.
- Summary
- Abstract
- Description
- Claims
- Application Information
AI Technical Summary
Problems solved by technology
Method used
Image
Examples
Embodiment Construction
[0061] The generation network needs to be trained with massive data to generate realistic image data, and the gradient during the training process or the leakage of the network weight after the training may allow the attacker to guess whether a certain data is training or not through model inference attacks or member inference attacks. data. Therefore, in order to ensure the privacy of the training process of the generative model, the generative network consists of two parts, namely the discriminator and the generator. Both are neural networks, so the training process is similar, roughly inputting the training data into the network to obtain the result, calculating the loss function based on the result, and deriving the loss function to obtain the gradient of the discriminator or generator. According to the post-processing nature of differential privacy, as long as the training process of discriminator meets differential privacy protection, the training process of generator na...
PUM
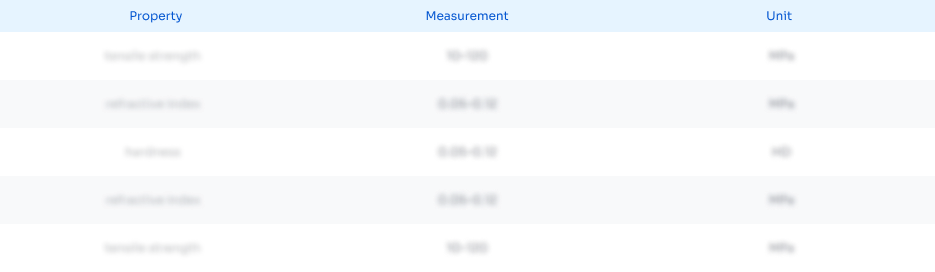
Abstract
Description
Claims
Application Information
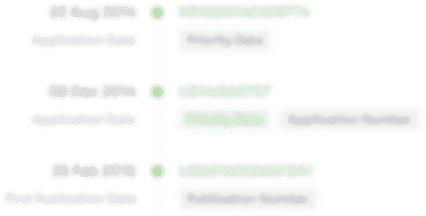
- R&D
- Intellectual Property
- Life Sciences
- Materials
- Tech Scout
- Unparalleled Data Quality
- Higher Quality Content
- 60% Fewer Hallucinations
Browse by: Latest US Patents, China's latest patents, Technical Efficacy Thesaurus, Application Domain, Technology Topic, Popular Technical Reports.
© 2025 PatSnap. All rights reserved.Legal|Privacy policy|Modern Slavery Act Transparency Statement|Sitemap|About US| Contact US: help@patsnap.com