Battery module safety state evaluation method and device based on convolutional neural network
A technology of convolutional neural network and safety state assessment, applied in biological neural network models, neural learning methods, measurement devices, etc., can solve the problem of not being able to identify thermal runaway or thermal runaway battery cells, and not being able to evaluate the safety state of battery cells at the same time and health status, the failure to detect the overall risk of the energy storage battery module in time, etc., to achieve the effect of low misjudgment rate and high accuracy
- Summary
- Abstract
- Description
- Claims
- Application Information
AI Technical Summary
Problems solved by technology
Method used
Image
Examples
Embodiment 1
[0054] like figure 1 As shown, a method for evaluating the safety status of a battery module based on a convolutional neural network, the method includes:
[0055] S1: Extract the output voltage, output current and surface temperature at the center of the battery cell under different decay states and different working states, form a three-parameter collaborative evaluation database, and divide the samples in the database into a training set and a test set; the specific process is as follows:
[0056] The state of health (SOH) of a battery cell is equal to the current power after the battery is cycled and the battery capacity before the battery cell is cycled. For multiple groups of lithium-ion batteries, cycle between the discharge cut-off voltage and the charge cut-off voltage for many times by means of constant current and constant voltage charging or constant current discharge through standard charging or discharging rates, until different health states (100% SOH, 85% SOH,...
Embodiment 2
[0081] The invention also provides a battery module safety state assessment device based on a convolutional neural network, comprising a data acquisition module, a data processing module and a display module connected in sequence,
[0082] The data acquisition module is used to collect the output voltage signal, the output current signal and the temperature signal at the center wall of the battery unit, and send the collection result to the data processing module;
[0083] a data processing module, configured to receive the data of the data acquisition module, and execute the method described in any of the above;
[0084] The display module is used for displaying the processing and classification results of the data processing module on the front end of the computer.
[0085] Specifically, the data acquisition module includes a SMD thermistor and a BMS battery management system. The SMD thermistor is attached to the central wall of the battery unit of the battery module, and i...
PUM
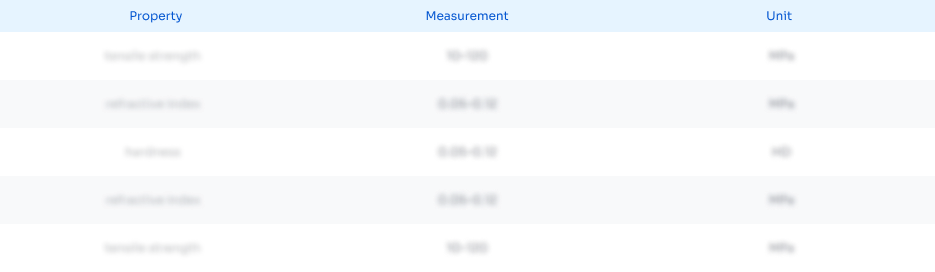
Abstract
Description
Claims
Application Information
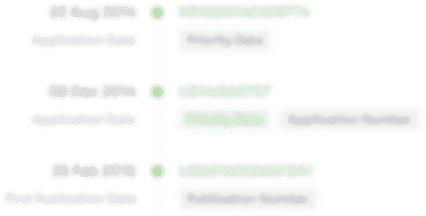
- R&D Engineer
- R&D Manager
- IP Professional
- Industry Leading Data Capabilities
- Powerful AI technology
- Patent DNA Extraction
Browse by: Latest US Patents, China's latest patents, Technical Efficacy Thesaurus, Application Domain, Technology Topic, Popular Technical Reports.
© 2024 PatSnap. All rights reserved.Legal|Privacy policy|Modern Slavery Act Transparency Statement|Sitemap|About US| Contact US: help@patsnap.com