CNN multi-information fusion-based GNSS-R sea surface wind speed inversion method and system
A technology of multi-information fusion and sea surface wind speed, applied in neural learning methods, ICT adaptation, climate sustainability, etc., can solve the problems of DDM feature loss of effective information, difficult to consider GNSS-R wind speed retrieval process information, etc., to achieve High training and use efficiency, high precision, and comprehensive effects
- Summary
- Abstract
- Description
- Claims
- Application Information
AI Technical Summary
Problems solved by technology
Method used
Image
Examples
Embodiment 1
[0041] Embodiment 1 of the present invention provides a GNSS-R sea surface wind speed inversion method based on CNN multi-information fusion, which uses convolutional neural network to extract DDM features, and then uses the latitude and longitude, RCG, signal incident angle, and effective wave height of the specular reflection point. Feature fusion, input wind speed inversion model, output inversion wind speed, the realization process includes the following steps:
[0042] Step S1: constructing the DDM and the convolution input vector corresponding to the effective scattering area and the auxiliary vector of the longitude and latitude, RCG, signal incident angle, and effective wave height of the specular reflection point;
[0043] Further, step S1 may specifically include:
[0044] Step S1.1: Match the GNSS-R data with the effective wave height data in space and time;
[0045] Step S1.2: Combine the GNSS-R L1b BRCS DDM and the effective scattering cross-sectional area of t...
Embodiment 2
[0065] Embodiment 2 of the present invention provides a GNSS-R sea surface wind speed inversion method based on CNN multi-information fusion, which can also be implemented using this process, which mainly includes the following steps:
[0066] The first step is to download and save the dataset. Use python script to batch download CYGNSS L1 data (CYGNSS data is a kind of spaceborne GNSS-R data), ECMWF wind speed data and ECMWF effective wave height data of the specified date range, and save them to the specified database;
[0067] The second step is data preprocessing and data set matching. Calculate the RCG in the data sample corresponding to CYNGSS and write it to the file, and interpolate and match the CYGNSS data with the wind speed data and effective wave height according to space and time in turn;
[0068] The third step is to generate training and test sets. According to the preset data screening conditions, filter the matched CYGNSS data set, and then divide the train...
Embodiment 3
[0106] In another embodiment 3 of the present invention, a GNSS-R sea surface wind speed inversion system based on CNN multi-information fusion is also provided, such as image 3 shown, including:
[0107] The data processing module is used to calculate the RCG and perform space-time matching between the CYGNSS data and the ECMWF significant wave height data;
[0108] Model storage module, used to store the trained CNN model;
[0109] The wind speed inversion module inputs the processed CYGNSS data into the CNN model for wind speed inversion, and gives the corresponding inversion performance.
[0110] Further, the wind speed inversion module specifically includes:
[0111] Combine the BRCS DDM in the GNSS-R data to be inverted and the effective scattering cross-sectional area of the corresponding size into the input vector of the convolution layer, and normalize it;
[0112] Combine the longitude and latitude, RCG, signal incident angle, and effective wave height of the s...
PUM
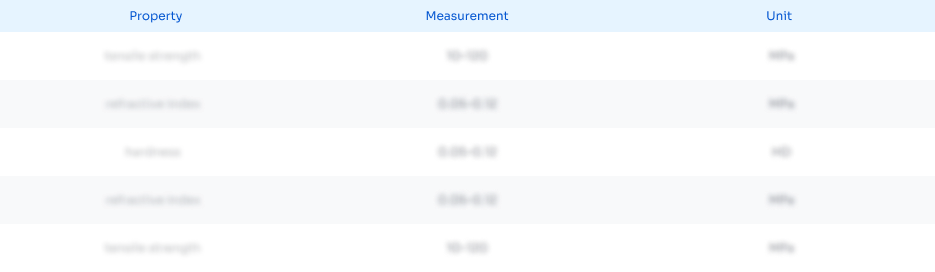
Abstract
Description
Claims
Application Information
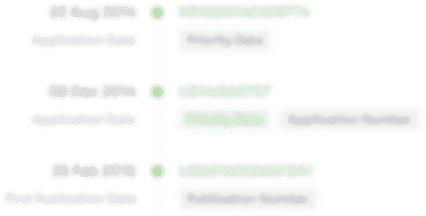
- R&D Engineer
- R&D Manager
- IP Professional
- Industry Leading Data Capabilities
- Powerful AI technology
- Patent DNA Extraction
Browse by: Latest US Patents, China's latest patents, Technical Efficacy Thesaurus, Application Domain, Technology Topic, Popular Technical Reports.
© 2024 PatSnap. All rights reserved.Legal|Privacy policy|Modern Slavery Act Transparency Statement|Sitemap|About US| Contact US: help@patsnap.com