Self-adapting image compressive sampling method based on multi-dimension saliency map
An image compression, multi-scale technology, applied in the field of image processing, can solve the problem of waste of sampling resources, to avoid the effect of reducing the size
- Summary
- Abstract
- Description
- Claims
- Application Information
AI Technical Summary
Problems solved by technology
Method used
Image
Examples
Embodiment 1
[0049] refer to figure 1 , the present invention is an adaptive image compression sampling method based on a multi-scale saliency map, which can be used in a single-pixel image acquisition system, and the method includes the following steps:
[0050] (1) Obtain a low-resolution sampling image P through acquisition by a low-resolution sensor;
[0051] (2) Perform SVT transformation on the low-resolution sampling image P. When the decomposition level is set to 3, a low-frequency image P is obtained. 1 and three high-frequency support value images S 1 , S 2 , S 3 ;
[0052] Among them, the SVT transformation is carried out as follows:
[0053] 2a) Given the size of the rectangular neighborhood of the mapped input vector space, that is, the rectangular neighborhood of N×N size, given the parameter γ of the least squares vector machine and the parameter σ of the Gaussian radial basis (RBF) kernel function, the above The values of the parameters are all empirical values. Th...
Embodiment 2
[0079] The adaptive image compression sampling method based on the multi-scale saliency map is the same as that in Embodiment 1. In order to describe the method of the present invention in detail, the implementation steps and conditions of the method are fused together and explained as follows:
[0080] Step 1. Low resolution sampling:
[0081] A low-resolution image P obtained by a low-resolution sensor.
[0082]Step 2. Use SVT to transform the low-resolution sampled image P to obtain a saliency map:
[0083] 2a) Given a rectangular neighborhood of N×N size mapped to the input vector space, given the parameter γ of the least squares vector machine and the parameter σ of the Gaussian Radial Basis (RBF) kernel function, all of the above are empirical parameters. The mathematical expression of the RBF kernel function is:
[0084] K(x,x i )=exp(-‖x-x i ‖ 2 / 2σ 2 )
[0085] Where x is the position vector of the image pixel, x i is the position vector of the i-th pixel of t...
Embodiment 3
[0110] The adaptive image compression sampling method based on the multi-scale saliency map is the same as the embodiment 1-2, and the effect of the present invention can be further illustrated by the following experiments:
[0111] 1) Experimental conditions
[0112] This experiment uses natural images, SAR images, and visible light remote sensing images as experimental data, and uses software MATLAB 7.9.0 as a simulation tool, and the computer configuration is Intel Core2 / 2.13G / 2G.
[0113] 2) Experimental content
[0114] Firstly, the saliency map generation model is used to obtain the saliency map for the low-resolution image, the "visually salient" area and the "non-visually salient" area of the image are determined, and then the number of measurements is allocated. Through the OMP reconstruction algorithm, the reconstructed image is finally obtained.
[0115] In this experiment, a random Gaussian matrix is selected as the observation matrix, the average sampling rat...
PUM
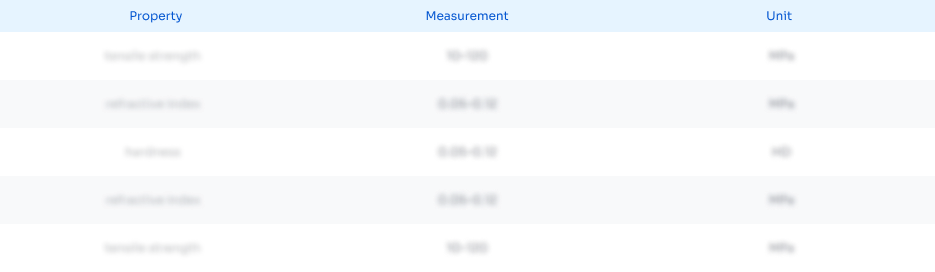
Abstract
Description
Claims
Application Information
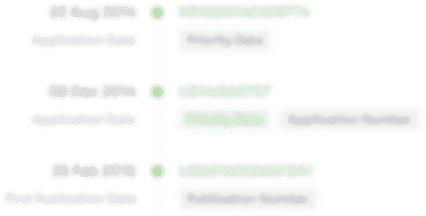
- R&D Engineer
- R&D Manager
- IP Professional
- Industry Leading Data Capabilities
- Powerful AI technology
- Patent DNA Extraction
Browse by: Latest US Patents, China's latest patents, Technical Efficacy Thesaurus, Application Domain, Technology Topic, Popular Technical Reports.
© 2024 PatSnap. All rights reserved.Legal|Privacy policy|Modern Slavery Act Transparency Statement|Sitemap|About US| Contact US: help@patsnap.com