A wta neural network based on memristor array and its application
A neural network and memristor technology, applied in the field of neural networks, can solve the problems of large size, high power consumption, and unfavorable large-scale use of neural networks, and achieve the effects of small size, low power consumption, and excellent performance.
- Summary
- Abstract
- Description
- Claims
- Application Information
AI Technical Summary
Problems solved by technology
Method used
Image
Examples
Embodiment 1
[0030] Use 12 memristors, 12 MOS tubes, 4 capacitors, 4 resistors, and 4 DC power supplies to connect according to the above-mentioned connection method, that is, to construct a memristor-based with four neurons Array of WTA neural networks, such as figure 2 Shown. Analyzing the network, the dynamic equation (4) can be obtained, where N=4. Through mathematical analysis of the dynamic equation, it can be verified that the neural network has WTA characteristics.
Embodiment 2
[0032] Use 56 memristors, 56 MOS tubes, 8 capacitors, 8 resistors, and 8 DC power supplies to connect according to the above-mentioned connection method, that is, to construct a memristor-based with eight neurons Array of WTA neural network. Analyzing the network, the dynamic equation formula (4) can be obtained, where N=8. Through mathematical analysis of the dynamic equation, it can be verified that the neural network has WTA characteristics.
[0033] Finally, the MATLAB simulations of Example 1 and Example 2 were performed respectively, and the simulation results were as follows: image 3 , Figure 4 Shown. It can be seen from the simulation results that only one output is positive and the other outputs are negative, showing the WTA characteristics.
Embodiment 3
[0035] The WTA neural network classifier based on the memristor array combines the BP neural network with the WTA neural network based on the memristor array, which is connected through a converter. After the output of the first part of the BP network is isolated and driven by the second part of the converter, it is used as the input of the third part of the WTA neural network. Combine the above-mentioned embodiment 1 to construct a classifier, its structure is as follows Figure 5 Shown.
[0036] In order to verify the effect of the classifier, we apply it to the classification and recognition of skin diseases. We use the data in the Dermatology Database to classify the sub-types of skin diseases such as erythemato-scuamous. We used 366 groups of data: 72 groups were lichen planus (lichen planus), 49 groups were pityriasis rosea (pityriasis rosea), 52 groups were chronic dermatitis (chronic dermatitis), 112 groups were psoriasis (psoriasis), 61 groups were Seborrheic dermatiti...
PUM
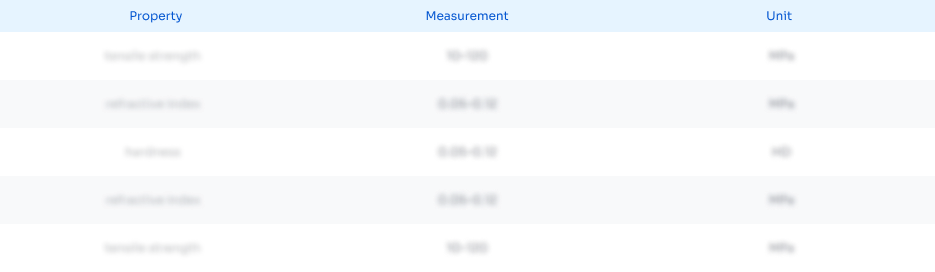
Abstract
Description
Claims
Application Information
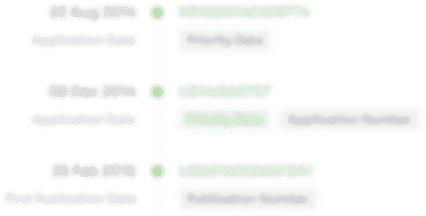
- Generate Ideas
- Intellectual Property
- Life Sciences
- Materials
- Tech Scout
- Unparalleled Data Quality
- Higher Quality Content
- 60% Fewer Hallucinations
Browse by: Latest US Patents, China's latest patents, Technical Efficacy Thesaurus, Application Domain, Technology Topic, Popular Technical Reports.
© 2025 PatSnap. All rights reserved.Legal|Privacy policy|Modern Slavery Act Transparency Statement|Sitemap|About US| Contact US: help@patsnap.com