Optimal Latin hypercube design (LHD) method based on adaptive genetic algorithm
A Latin hypercube, experimental design technology, applied in the fields of genetic law, calculation, genetic model, etc., can solve the problems of high optimization cost, premature convergence, falling into local optimal solution, etc., to achieve high sampling efficiency, improve optimization accuracy, Test point characteristic good effect
- Summary
- Abstract
- Description
- Claims
- Application Information
AI Technical Summary
Problems solved by technology
Method used
Image
Examples
specific Embodiment approach 1
[0024] Specific implementation mode one: as figure 1 and figure 2 As shown, an optimal Latin hypercube experiment design method based on adaptive genetic algorithm includes the following steps:
[0025] Step 1: Randomly generate n Latin hypercube experimental design matrices LHD(N, P), where N is the number of experiments, P is the number of experimental factors, and each LHD(N, P) is a matrix of order N×P, which is recorded as a matrix L; the initial population N consisting of n LHD(N, P) individuals pop Expressed as a matrix of k×N, where k=n×P;
[0026] Step 2: Determine the fitness function of the population individual in the optimization process, and calculate the population N pop The fitness value of the n individuals in the middle, and select the individual with the largest fitness value as the current optimal LHD(N, P);
[0027] Step 3: Sort the n fitness values calculated in step 2 from large to small, select the first n / 2 individuals as parents, and the rest a...
specific Embodiment approach 2
[0030] Specific embodiment 2: The difference between this embodiment and specific embodiment 1 is that the column vector of matrix L in the step 1 represents the value of the test factor, and each column is arranged arbitrarily from 1 to N, and the matrix L is arbitrarily arranged. line x i =[x i1 ,x i2 ,...,x iP ], which is a sample point.
[0031] The randomly generated sample size is 6, and the schematic diagram of the Latin hypercube experimental design with a factor number of 2 is LHD (6, 2). figure 2 As shown, the Latin hypercube experimental design matrix L is:
[0032]
[0033] Other steps and parameters are the same as those in Embodiment 1.
specific Embodiment approach 3
[0034] Specific embodiment three: the difference between this embodiment and specific embodiment one or two is: the specific process of determining the fitness function of the population individual in the optimization process in the step two is:
[0035] Firstly, the Audze–Eglais criterion is chosen to describe the property of "filled space". The AE criterion can effectively avoid the defect that the genetic algorithm itself is easy to fall into the local optimum, and can be effectively combined with the genetic algorithm.
[0036] Select the optimization objective function f(x)=G(L), and the fitness function is:
[0037]
[0038] where c max is the maximum estimated value of f(x);
[0039]
[0040] Where G(L) represents the potential energy of a LHD(N, P), ||x i -x j || represents the distance between any two rows in LHD(N, P). x j =[x j1 ,x j2 ,...,x jP ] to distinguish from x i Another sample point of .
[0041] Other steps and parameters are the same as th...
PUM
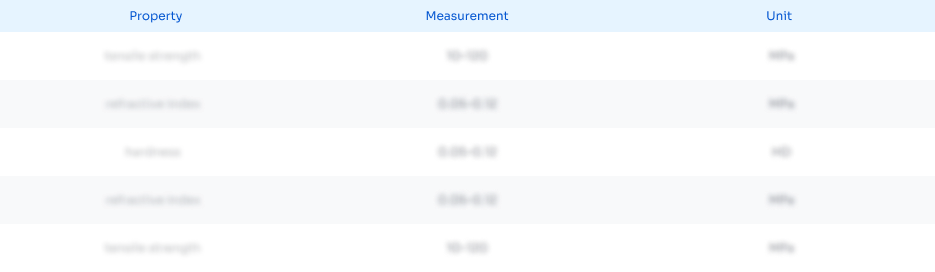
Abstract
Description
Claims
Application Information
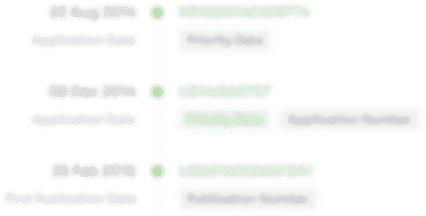
- R&D Engineer
- R&D Manager
- IP Professional
- Industry Leading Data Capabilities
- Powerful AI technology
- Patent DNA Extraction
Browse by: Latest US Patents, China's latest patents, Technical Efficacy Thesaurus, Application Domain, Technology Topic, Popular Technical Reports.
© 2024 PatSnap. All rights reserved.Legal|Privacy policy|Modern Slavery Act Transparency Statement|Sitemap|About US| Contact US: help@patsnap.com