Hyperspectral image classification method based on spatial information enhancement and deep belief network
A deep belief network and hyperspectral image technology, applied in the field of hyperspectral image classification, can solve the problems of spatial information enhancement, affecting original spectral information, spectral confusion, etc., achieve high classification accuracy and enhance the effect of spatial information
- Summary
- Abstract
- Description
- Claims
- Application Information
AI Technical Summary
Problems solved by technology
Method used
Image
Examples
Embodiment Construction
[0028] The embodiments and effects of the present invention will be further described below in conjunction with the accompanying drawings.
[0029] refer to figure 1 , the present invention realizes steps as follows:
[0030] Step 1, preprocessing the original hyperspectral image:
[0031] (1a) Normalization:
[0032] Let X ∈ R M×N×L Represents an original hyperspectral image, where M represents the length of X, N represents the width of X, and L represents the number of bands of X;
[0033] The hyperspectral image X contains M×N spectral vectors of length L, the reflectance values of these M×N spectral vectors are normalized, and the reflectance values of each spectral vector are normalized in [0- 1] to get the normalized hyperspectral image X u ,in:
[0034] Each spectral vector is normalized using the following formula:
[0035]
[0036] where X r Represents the rth spectral vector of the hyperspectral image, Max(X r ) represents the spectral vector X r The...
PUM
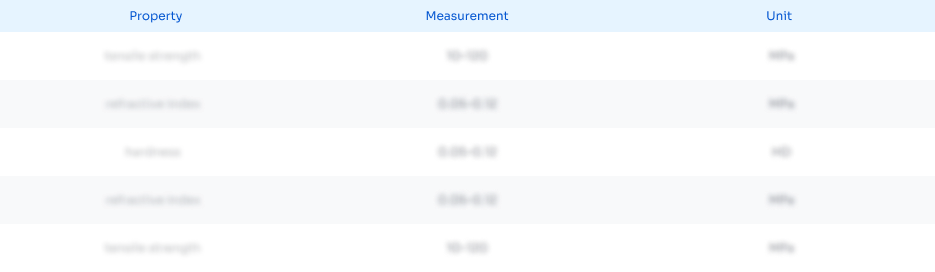
Abstract
Description
Claims
Application Information
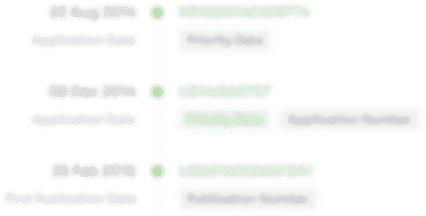
- R&D Engineer
- R&D Manager
- IP Professional
- Industry Leading Data Capabilities
- Powerful AI technology
- Patent DNA Extraction
Browse by: Latest US Patents, China's latest patents, Technical Efficacy Thesaurus, Application Domain, Technology Topic, Popular Technical Reports.
© 2024 PatSnap. All rights reserved.Legal|Privacy policy|Modern Slavery Act Transparency Statement|Sitemap|About US| Contact US: help@patsnap.com