MIMU gyroscope random drift forecasting method based on ARMA and BPNN combination model
A technology of random drift and combined models, applied in neural learning methods, biological neural network models, measurement devices, etc., can solve problems such as filtering out useful signals, improper selection of wavelet layers, easy leakage of identification noise, etc. Modulo error, the effect of improving prediction performance
- Summary
- Abstract
- Description
- Claims
- Application Information
AI Technical Summary
Problems solved by technology
Method used
Image
Examples
Embodiment Construction
[0022] The present invention describes a predictive modeling method for MIMU gyroscope random errors. The invention adopts the predictive fitting algorithm of ARMA and BP neural network combination model. In order to obtain a more accurate gyroscope random drift model, the single ARMA model and BP The neural network cannot fully express the shortcomings of linear and nonlinear relationships. The proposed model prediction method is suitable for MIMU, and can greatly reduce the model prediction error and improve the accuracy of the model prediction. The design scheme of the present invention is as figure 1 As shown, the steps are as follows:
[0023] Step 1: Preprocess the real-time output data of the MIMU gyroscope. The random drift data of the single-axis gyroscope is collected in a static state, the stationarity of the original data sequence is judged, the correlation test is carried out on the test data of the gyroscope, and the order of the ARMA model is preliminarily dete...
PUM
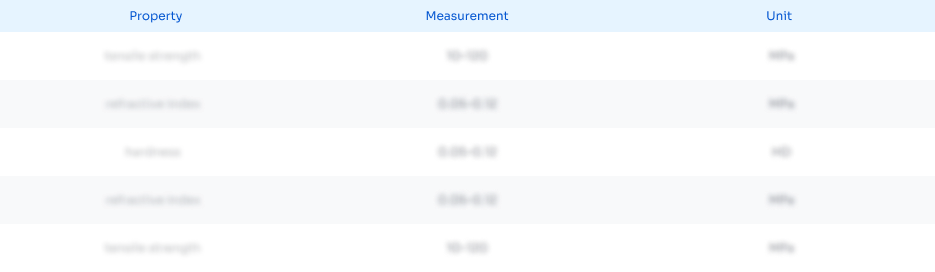
Abstract
Description
Claims
Application Information
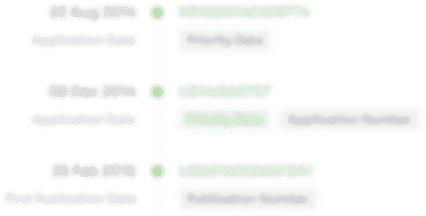
- R&D Engineer
- R&D Manager
- IP Professional
- Industry Leading Data Capabilities
- Powerful AI technology
- Patent DNA Extraction
Browse by: Latest US Patents, China's latest patents, Technical Efficacy Thesaurus, Application Domain, Technology Topic, Popular Technical Reports.
© 2024 PatSnap. All rights reserved.Legal|Privacy policy|Modern Slavery Act Transparency Statement|Sitemap|About US| Contact US: help@patsnap.com