Intrusion detection method and intrusion detection system based on sustainable ensemble learning
An integrated learning and intrusion detection technology, applied in transmission systems, machine learning, instruments, etc., can solve the problems of sensitivity, denial of service, and weak adaptability without considering the different attack types of individual learners
- Summary
- Abstract
- Description
- Claims
- Application Information
AI Technical Summary
Problems solved by technology
Method used
Image
Examples
Embodiment Construction
[0060] In order to make the objectives, technical solutions and advantages of the present invention clearer, the present invention will be further described in detail below with reference to the embodiments. It should be understood that the specific embodiments described herein are only used to explain the present invention, but not to limit the present invention.
[0061] The invention ensures that each individual learner can fully exert its adaptability, and at the same time, the historical model is added to the training and detection process of the new model in the detection model update stage, so as to complete the transfer of knowledge in the update process, so that the learning process becomes sustainable. , which further improves the stability and accuracy of the model.
[0062] The application principle of the present invention will be described in detail below with reference to the accompanying drawings.
[0063] like figure 1 As shown, the intrusion detection metho...
PUM
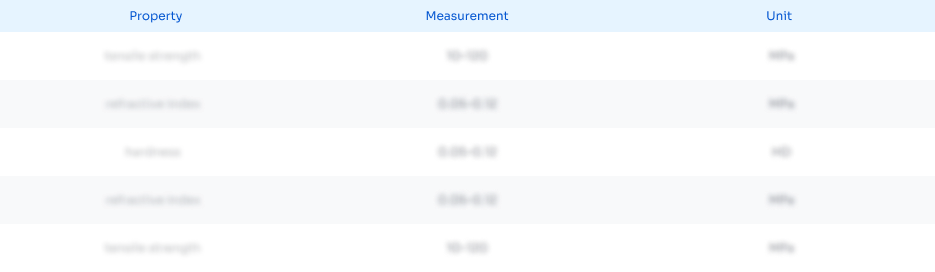
Abstract
Description
Claims
Application Information
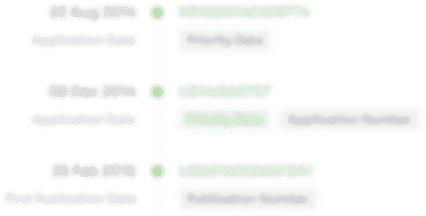
- R&D Engineer
- R&D Manager
- IP Professional
- Industry Leading Data Capabilities
- Powerful AI technology
- Patent DNA Extraction
Browse by: Latest US Patents, China's latest patents, Technical Efficacy Thesaurus, Application Domain, Technology Topic, Popular Technical Reports.
© 2024 PatSnap. All rights reserved.Legal|Privacy policy|Modern Slavery Act Transparency Statement|Sitemap|About US| Contact US: help@patsnap.com