Classification and grading of orthopedic disease lesions based on deep residual network
A classification method and technology for orthopedic diseases, applied in the field of classification and grading of orthopedic diseases based on deep residual network, can solve the problems of offline learning method, such as inability to use diagnosis and treatment data, decrease in accuracy due to saturation, and inability to correct, and achieve breakthrough in accuracy bottleneck. , reduce stress, improve the effect of precision
- Summary
- Abstract
- Description
- Claims
- Application Information
AI Technical Summary
Problems solved by technology
Method used
Image
Examples
Embodiment
[0083]After a total of 60,000 images of lumbar disc herniation, frozen shoulder, knee injury, and cervical spondylosis images classified by experts, the accuracy rate test was performed on 14,000 test data, and a 50-layer deep residual neural network was used. Under the framework, its accuracy can reach 97.8%. By simulating online learning, after 2000 error images were corrected, after online learning training, the accuracy rate rose to 98.2%. Higher than similar judgment accuracy using convolutional neural network.
[0084] In the offline learning part, this example is based on an open source deep learning framework, which consists of 50 layers of deep residual neural network units. The first layer is the input layer, and the middle hidden layer is 48 layers. The hidden layer includes a convolutional layer and a pooling layer. , the fully connected layer, and the last layer is the Soft-Max layer. There is a mapping channel between the convolutional layer and the convolution...
PUM
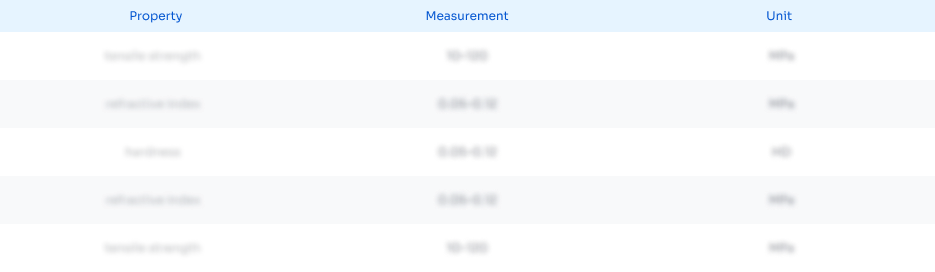
Abstract
Description
Claims
Application Information
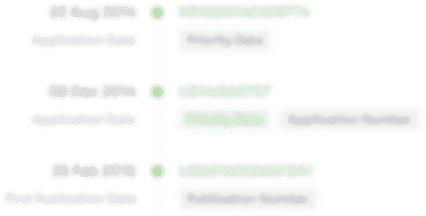
- R&D Engineer
- R&D Manager
- IP Professional
- Industry Leading Data Capabilities
- Powerful AI technology
- Patent DNA Extraction
Browse by: Latest US Patents, China's latest patents, Technical Efficacy Thesaurus, Application Domain, Technology Topic, Popular Technical Reports.
© 2024 PatSnap. All rights reserved.Legal|Privacy policy|Modern Slavery Act Transparency Statement|Sitemap|About US| Contact US: help@patsnap.com