Adaptive Optimal Scheduling Method for Mobile Terminal Software Based on Deep Reinforcement Learning
A mobile terminal and reinforcement learning technology, applied in the computing field, can solve problems such as overshooting, increasing antenna transmission power loss, and long unloading time, so as to optimize process scheduling and unloading, improve user experience, and reduce energy consumption.
- Summary
- Abstract
- Description
- Claims
- Application Information
AI Technical Summary
Problems solved by technology
Method used
Examples
Embodiment Construction
[0025] The technical solution of the present invention is further described below in conjunction with the examples, but the scope of protection is not limited to the description.
[0026] Embodiments of the present invention include the following steps:
[0027] Step 1: The mobile terminal device is connected to the surrounding edge computing devices through the wireless network.
[0028]Step 2: Construct a deep convolutional neural network with 4 layers. The first layer is a convolutional layer, the number of inputs is 21×21, it contains 20 convolution kernels of 10×10, the step is 1, and the number of outputs is 20×12×12; the second layer is a convolutional layer , the number of inputs is 20×12×12, including 40 convolution kernels of 5×5, the step is 1, and the number of outputs is 40×8×8; the third layer is a fully connected layer, and the number of inputs is 2560, the number of outputs is 1024; the last layer is a fully connected layer, the input size is 1024, and the nu...
PUM
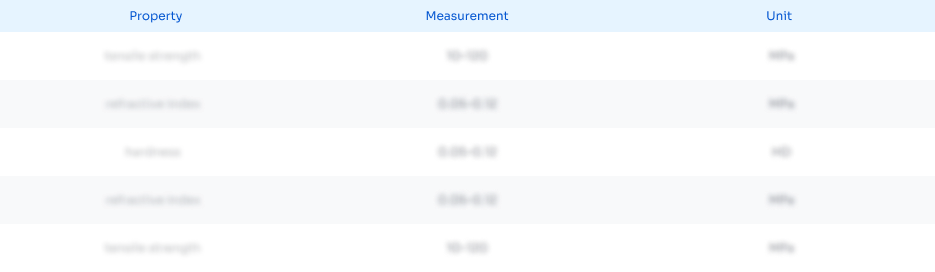
Abstract
Description
Claims
Application Information
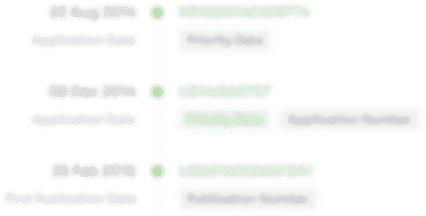
- R&D Engineer
- R&D Manager
- IP Professional
- Industry Leading Data Capabilities
- Powerful AI technology
- Patent DNA Extraction
Browse by: Latest US Patents, China's latest patents, Technical Efficacy Thesaurus, Application Domain, Technology Topic, Popular Technical Reports.
© 2024 PatSnap. All rights reserved.Legal|Privacy policy|Modern Slavery Act Transparency Statement|Sitemap|About US| Contact US: help@patsnap.com