A short-term wind power prediction method based on double-time sequence feature learning
A wind power prediction and feature learning technology, applied in complex mathematical operations, biological neural network models, instruments, etc., to achieve the effects of improving prediction accuracy, learning ability, and robustness
- Summary
- Abstract
- Description
- Claims
- Application Information
AI Technical Summary
Problems solved by technology
Method used
Image
Examples
Embodiment 1
[0033] A short-term wind power prediction method based on dual time series feature learning, the method is based on the dual time series feature learning neural network model (BTFLNN), the key points of its prediction technology are: 1) effective data denoising; 2) sufficient time series features extract.
[0034] The main design and analysis process of the entire BTFLNN model can be divided into the following four parts: 1) data selection and conversion; 2) data denoising and principal component selection; 3) dual time series feature learning of data and wind power regression analysis; 4) BTFLNN model prediction results analysis.
[0035] The method includes the following steps:
[0036] 101: Construct a training set and a test set, and simultaneously convert the original data into labeled data;
[0037] 102: Use the singular spectrum analysis method to de-dry and select the principal components of the labeled data;
[0038] 103: Construct a dual time-series feature learni...
Embodiment 2
[0042] The following is combined with specific examples, mathematical formulas, Figure 1-Figure 3 , the scheme in embodiment 1 is further introduced, see the following description for details:
[0043] 1. Data selection and conversion
[0044] The experimental data set in the embodiment of the present invention comes from a wind farm in Chenzhou City, Hunan Province, China. All data of fans No. 26 to No. 50. Specifically, the installed capacity of these 25 wind turbines is 2000KW, and the time resolution of the data is 10 minutes. The collected data contains five variables, namely: average temperature outside the engine room, reactive power, active power, wind speed and wind direction . Since the reactive power is an irrelevant variable (not beneficial to the design and realization of the model), this variable is removed, leaving the remaining four relevant variables. In addition, in actual scenarios, it is a very common phenomenon that wind turbines are disconnected from...
Embodiment 3
[0084] Combine below Figure 4-Figure 8 The scheme in embodiment 1 and 2 is carried out feasibility verification, see the following description for details:
[0085] This experiment uses two evaluation indicators commonly used in data prediction experiments: mean absolute error (NMAE) and root mean square error (NRMSE) to verify the prediction performance of the dual temporal feature learning neural network model BTFLNN. Among them, NMAE is a linear evaluation index, and NRMSE is a secondary evaluation index, which is more sensitive to errors. The calculation methods of NMAE and NRMSE are described as follows (the lower the value, the better the model effect):
[0086]
[0087]
[0088] Among them, p inst is the installed capacity of the fan, N is the length of the time series data, x' n is the predicted value, x n is the actual measured value.
[0089] Figure 4 It shows the corresponding NMAE and NRMSE results when the input data of the model takes different leng...
PUM
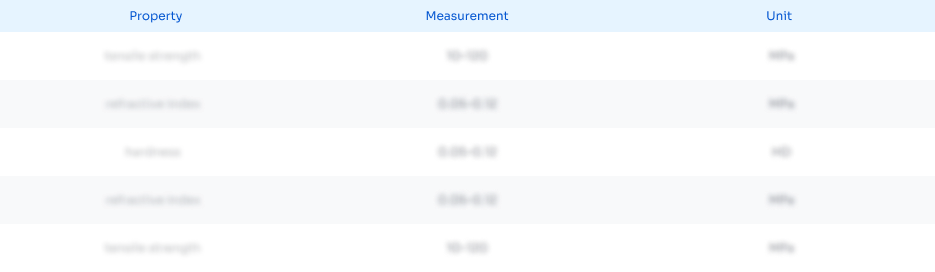
Abstract
Description
Claims
Application Information
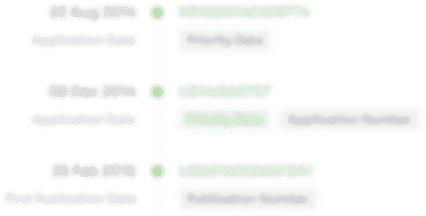
- R&D Engineer
- R&D Manager
- IP Professional
- Industry Leading Data Capabilities
- Powerful AI technology
- Patent DNA Extraction
Browse by: Latest US Patents, China's latest patents, Technical Efficacy Thesaurus, Application Domain, Technology Topic, Popular Technical Reports.
© 2024 PatSnap. All rights reserved.Legal|Privacy policy|Modern Slavery Act Transparency Statement|Sitemap|About US| Contact US: help@patsnap.com