Electric energy quality analysis method based on variational mode decomposition multi-scale permutation entropy
A technology of variational mode decomposition and power quality monitoring, applied in neural learning methods, biological neural network models, instruments, etc., can solve problems such as difficult real-time online measurement of power quality, noise sensitivity, lack of adaptability, etc.
- Summary
- Abstract
- Description
- Claims
- Application Information
AI Technical Summary
Problems solved by technology
Method used
Image
Examples
Embodiment Construction
[0065] The following is a detailed description of the embodiments of the present invention. This embodiment is carried out based on the technical solution of the present invention, and provides detailed implementation methods and specific operation processes to further explain the technical solution of the present invention.
[0066] Such as figure 1 As shown, the present invention provides a power quality analysis method based on variational mode decomposition multi-scale permutation entropy. By extracting the multi-frequency band and multi-scale permutation entropy information of electrical signals, the neural network classification model is used to realize real-time monitoring and analysis of power quality. Fault type judgment. The main content includes the following steps:
[0067] Step 1, collect the original training data of the power quality monitoring points when they are subjected to different types of disturbances;
[0068] Collect the voltage signal U of the power...
PUM
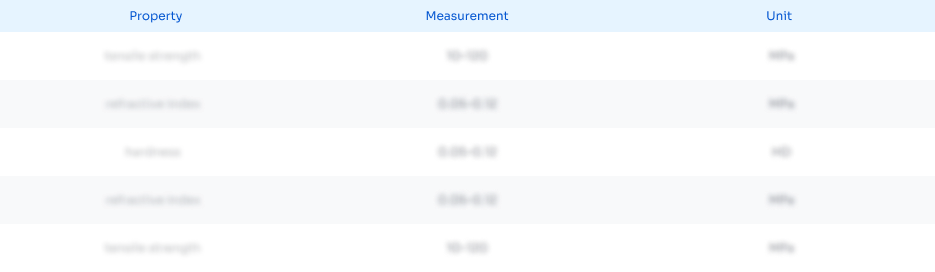
Abstract
Description
Claims
Application Information
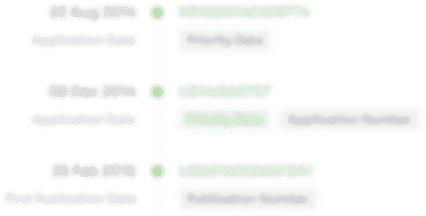
- R&D Engineer
- R&D Manager
- IP Professional
- Industry Leading Data Capabilities
- Powerful AI technology
- Patent DNA Extraction
Browse by: Latest US Patents, China's latest patents, Technical Efficacy Thesaurus, Application Domain, Technology Topic, Popular Technical Reports.
© 2024 PatSnap. All rights reserved.Legal|Privacy policy|Modern Slavery Act Transparency Statement|Sitemap|About US| Contact US: help@patsnap.com