Motor imagery identification method and system fusing CNN-BiLSTM model and probability cooperation
A technology of motor imagery and recognition methods, applied in character and pattern recognition, neural learning methods, biological neural network models, etc.
- Summary
- Abstract
- Description
- Claims
- Application Information
AI Technical Summary
Problems solved by technology
Method used
Image
Examples
Embodiment 1
[0076] as attached figure 1 As shown, the motion imagery recognition method of the fusion CNN-BiLSTM model and probability cooperation of the present invention, this method captures and extracts the spatio-temporal depth feature in the EEG signal by the CNN model of fusion BiLSTM network, and the time of capturing and extracting - Input the empty depth feature to the ProCRC classifier for classification, and use the test set data to evaluate the performance of the built CNN-BiLSTM model to realize the user's intention recognition; the specific steps are as follows:
[0077] S1. Collecting EEG signals; wherein, the EEG signal is a non-invasive EEG signal with weak signal amplitude, low signal-to-noise ratio, non-stationarity, nonlinearity, and great processing difficulty;
[0078] Dataset III (DatasetIVa) in the international BCI Competition III competition database was used in the experiment. Dataset IVa is a two-type motor imagery task involving the right hand and feet, and E...
Embodiment 2
[0131] as attached Image 6 As shown, the fusion CNN-BiLSTM model of the present invention and the motion imagery recognition system of probability cooperation, this system comprises,
[0132] The EEG signal acquisition unit is used to collect EEG signals; among them, the EEG signal is a non-invasive EEG signal, which has weak signal amplitude, low signal-to-noise ratio, non-stationarity, and nonlinearity, and is difficult to process ;
[0133] The deep neural network construction unit is used to extract the spatio-temporal depth features of the EEG signal through the convolutional neural network (CNN) in deep learning combined with the bidirectional long-short-term memory model network (BiLSTM);
[0134] Among them, the deep neural network construction unit includes,
[0135] The CNN model constructs a subunit for extracting spatial features in the EEG signal through a convolutional neural network;
[0136] The BiLSTM model construction subunit is used to construct a two-w...
PUM
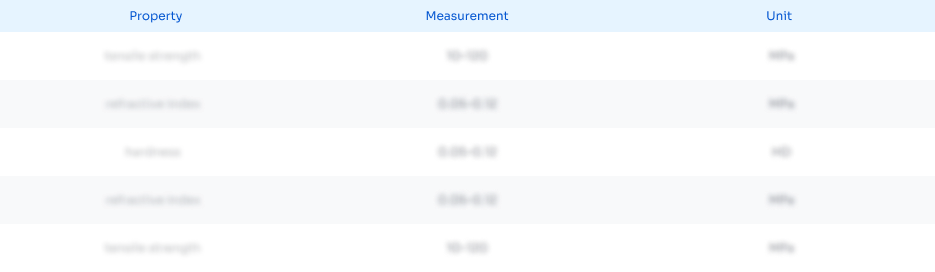
Abstract
Description
Claims
Application Information
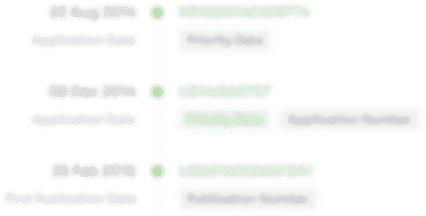
- R&D
- Intellectual Property
- Life Sciences
- Materials
- Tech Scout
- Unparalleled Data Quality
- Higher Quality Content
- 60% Fewer Hallucinations
Browse by: Latest US Patents, China's latest patents, Technical Efficacy Thesaurus, Application Domain, Technology Topic, Popular Technical Reports.
© 2025 PatSnap. All rights reserved.Legal|Privacy policy|Modern Slavery Act Transparency Statement|Sitemap|About US| Contact US: help@patsnap.com