Early warning method and device for NOx exceeding standard of gas turbine based on LSTM deep learning
A technology of deep learning and gas turbine, applied in the direction of instrumentation, adaptive control, control/regulation system, etc., can solve problems such as large prediction error, control influence, large detection data value, etc., achieve high accuracy and avoid omission or error , the effect of collecting a large amount of data
- Summary
- Abstract
- Description
- Claims
- Application Information
AI Technical Summary
Problems solved by technology
Method used
Image
Examples
Embodiment 1
[0054] Such as Figure 1-3 As shown, a gas turbine NOx excessive early warning method based on LSTM deep learning is characterized in that it includes the following steps:
[0055] Step 1: Obtain the historical operation data of the gas turbine and preprocess the historical data;
[0056] Step 2: Divide the model training set and test set according to the preprocessed historical data, and based on the training set, construct an LSTM prediction model with NOx emissions as the output;
[0057] Step 3: Use the test set data to input the gas turbine NOx emission model, compare whether the deviation between the actual value of NOx emission and the predicted value is within a reasonable range, and judge whether the model is valid; if it is invalid, proceed to step 4; if it is valid, save the model and end ;
[0058] Step 4: Adjust the hyperparameters such as the number of network layers and learning rate of LSTM, and re-use the training data for modeling and prediction after adjus...
Embodiment 2
[0096] A gas turbine NOx excess warning device based on LSTM deep learning that installs the above method, including a memory that is electrically connected and stores a program that implements the above method, a processor, I / O equipment, and an alarm device, and the I / O equipment is connected to the installation of the gas turbine. Computers and / or networks of monitoring software to access and obtain real-time measurement point data.
[0097] The processor is connected to the hand-held user terminal through wireless transmission. Remote monitoring and early warning through handheld devices.
PUM
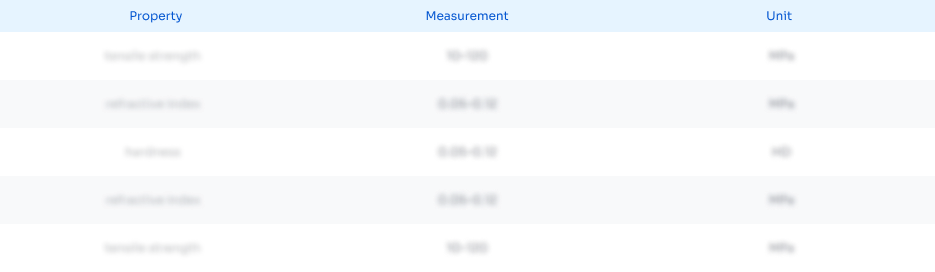
Abstract
Description
Claims
Application Information
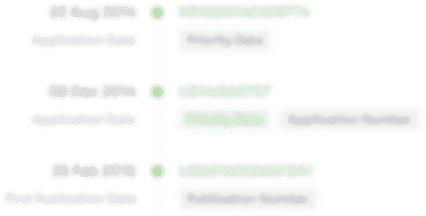
- R&D
- Intellectual Property
- Life Sciences
- Materials
- Tech Scout
- Unparalleled Data Quality
- Higher Quality Content
- 60% Fewer Hallucinations
Browse by: Latest US Patents, China's latest patents, Technical Efficacy Thesaurus, Application Domain, Technology Topic, Popular Technical Reports.
© 2025 PatSnap. All rights reserved.Legal|Privacy policy|Modern Slavery Act Transparency Statement|Sitemap|About US| Contact US: help@patsnap.com