Diagnosis model for industrial equipment fault diagnosis, construction method thereof and application thereof
A technology for industrial equipment and fault diagnosis, applied in data processing applications, instruments, computing, etc., can solve the problems of insufficient denoising of the original vibration signal, weak generalization ability, and insufficient practicability of industrial equipment fault diagnosis, and achieves good results. Noise reduction effect, high diagnostic accuracy, not easy to expand
- Summary
- Abstract
- Description
- Claims
- Application Information
AI Technical Summary
Problems solved by technology
Method used
Image
Examples
Embodiment 1
[0046] A diagnostic model construction method 100 for industrial equipment fault diagnosis, such as figure 1 shown, including:
[0047] Step 110, using the variational mode decomposition method to decompose each original vibration signal of the training industrial equipment to obtain multiple sub-modal components, and select an optimal sub-modal component from the multiple sub-modal components;
[0048] Step 120, using Bayesian optimized one-dimensional fast non-local mean method to denoise all optimal sub-mode components;
[0049] Step 130: Based on all denoised optimal sub-modal components, use metric learning to change the sample distance metric function in the classifier and train it. The trained classifier is a diagnostic model for industrial equipment fault diagnosis.
[0050] Since the fault features that are effective for the training model are often buried in useless noise such as noise, around the problem of noise reduction, considering that the variational mode dec...
Embodiment 2
[0080] A diagnostic model for fault diagnosis of industrial equipment, which is constructed by adopting any diagnostic model construction method for fault diagnosis of industrial equipment as described in the first embodiment above.
[0081] The relevant technical solutions are the same as those in Embodiment 1, and will not be repeated here.
[0082] The diagnostic model constructed by using any of the above diagnostic model construction methods for industrial equipment fault diagnosis has the characteristics of high accuracy, strong anti-noise performance and wide applicability due to the use of VMD, Bayesian optimized NLM and metric learning.
Embodiment 3
[0084] A method 200 for fault diagnosis of industrial equipment, such as image 3 shown, including:
[0085] Step 210, collecting the original vibration signal of the industrial equipment to be tested;
[0086] Step 220, using the variational mode decomposition method and the one-dimensional fast non-local mean method of Bayesian optimization as described in the first embodiment above, to decompose the original vibration signal to obtain the optimal sub-mode component and denoise;
[0087] Step 230, based on the optimal sub-modal component after denoising, use the diagnostic model described in the second embodiment above to diagnose and obtain the fault type of the industrial equipment to be tested.
[0088] The relevant technical solutions are the same as those in Embodiment 1 and Embodiment 2, and will not be repeated here.
[0089] Focusing on the problem of noise reduction, considering that VMD can decompose the signal into multiple intrinsic mode functions with limited ...
PUM
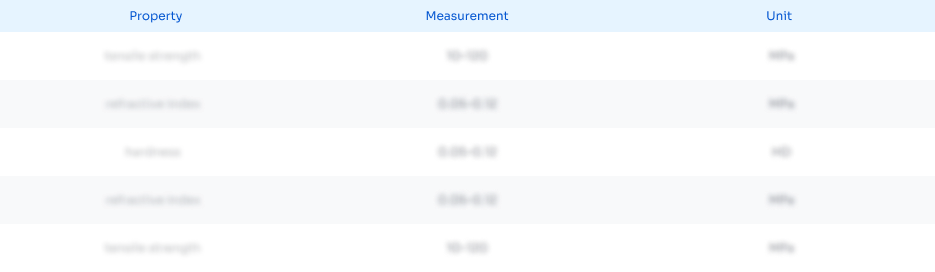
Abstract
Description
Claims
Application Information
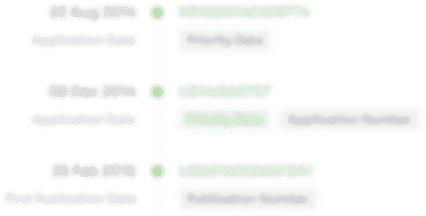
- R&D
- Intellectual Property
- Life Sciences
- Materials
- Tech Scout
- Unparalleled Data Quality
- Higher Quality Content
- 60% Fewer Hallucinations
Browse by: Latest US Patents, China's latest patents, Technical Efficacy Thesaurus, Application Domain, Technology Topic, Popular Technical Reports.
© 2025 PatSnap. All rights reserved.Legal|Privacy policy|Modern Slavery Act Transparency Statement|Sitemap|About US| Contact US: help@patsnap.com