Integrated circuit defect image recognition and classification system based on fusion deep learning model
A deep learning and image recognition technology, which is applied in image analysis, character and pattern recognition, image enhancement, etc., can solve the problems of low efficiency of manual recognition and low recognition rate of defect image categories, so as to reduce the cost of recognition, increase the level of automation, The effect of improving the yield rate
- Summary
- Abstract
- Description
- Claims
- Application Information
AI Technical Summary
Problems solved by technology
Method used
Image
Examples
Embodiment Construction
[0027] The technical solutions in the embodiments of the present invention will be clearly and completely described below in conjunction with the accompanying drawings in the embodiments of the present invention. Obviously, the described embodiments are only some of the embodiments of the present invention, not all of them. Based on the embodiments of the present invention, all other embodiments obtained by persons of ordinary skill in the art without making creative efforts belong to the protection scope of the present invention.
[0028] see Figure 1~3, in an embodiment of the present invention, an integrated circuit defect image recognition and classification system based on a fusion deep learning model is used as a neural network that simulates the process of processing visual images by the human brain. The convolutional network consists of a convolutional layer, a pooling layer, and a fully connected layer. composition. Among them, the convolutional layer cooperates wit...
PUM
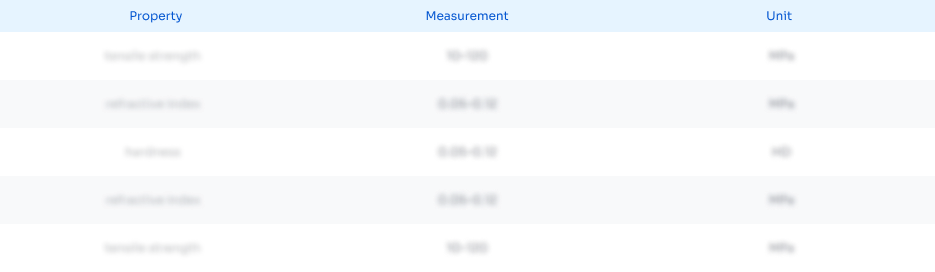
Abstract
Description
Claims
Application Information
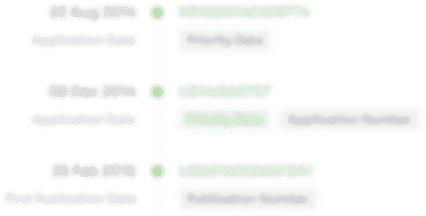
- R&D Engineer
- R&D Manager
- IP Professional
- Industry Leading Data Capabilities
- Powerful AI technology
- Patent DNA Extraction
Browse by: Latest US Patents, China's latest patents, Technical Efficacy Thesaurus, Application Domain, Technology Topic, Popular Technical Reports.
© 2024 PatSnap. All rights reserved.Legal|Privacy policy|Modern Slavery Act Transparency Statement|Sitemap|About US| Contact US: help@patsnap.com