2D + 3D large aircraft shape defect detection and analysis method based on deep learning
A defect detection and deep learning technology, applied in the fields of deep learning, computer vision and graphics, can solve problems such as tracking and positioning tasks that are not as direct as in vision, and sparse points, so as to achieve automatic application, accurate and effective detection and extraction, well thought out effects
- Summary
- Abstract
- Description
- Claims
- Application Information
AI Technical Summary
Problems solved by technology
Method used
Image
Examples
Embodiment Construction
[0048] This embodiment is a 2D+3D large aircraft shape defect detection and analysis method based on deep learning. The main content includes jointly learning 2D image and 3D point cloud feature point descriptors through Triplet (three-way) neural network; Euclidean distance similarity matrix between feature descriptors to obtain 2D-3D feature matching pairs; then, using the geometric relationship between 2D-3D matching pairs, the camera pose is estimated by the PnP method; using the estimated camera pose will The 3D point cloud is projected into the image space to obtain a textured 3D point cloud; self-supervised semantic segmentation is performed on the textured 3D point cloud; finally, defect analysis is performed on each component obtained from the semantic segmentation.
[0049] In order to further clarify the technical scheme and design principles of the present invention, the present invention will be described in detail below in conjunction with the accompanying drawing...
PUM
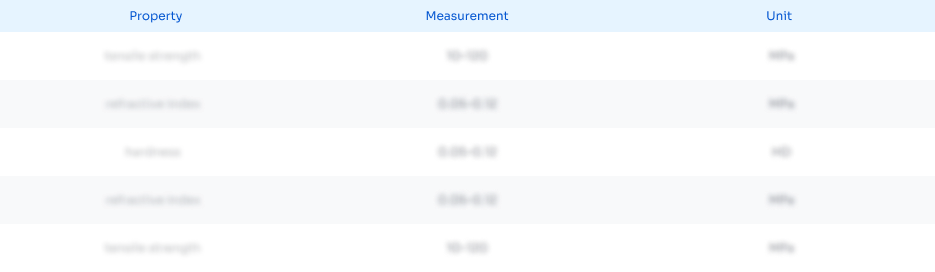
Abstract
Description
Claims
Application Information
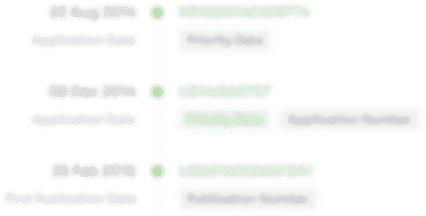
- R&D
- Intellectual Property
- Life Sciences
- Materials
- Tech Scout
- Unparalleled Data Quality
- Higher Quality Content
- 60% Fewer Hallucinations
Browse by: Latest US Patents, China's latest patents, Technical Efficacy Thesaurus, Application Domain, Technology Topic, Popular Technical Reports.
© 2025 PatSnap. All rights reserved.Legal|Privacy policy|Modern Slavery Act Transparency Statement|Sitemap|About US| Contact US: help@patsnap.com