Electrocardiogram classification method and device based on wavelet transform and DCNN (Deep Convolutional Neural Networks)
- Summary
- Abstract
- Description
- Claims
- Application Information
AI Technical Summary
Problems solved by technology
Method used
Image
Examples
Embodiment 1
[0064] see figure 1 , an electrocardiogram classification method based on wavelet transform and DCNN in the present invention, the method first adopts wavelet transform to filter the electrocardiogram signal, and locates the analysis on the time and frequency of the electrocardiogram signal, and through the stretching and translation operation, the signal is gradually Multi-scale refinement effectively retains the eigenvalues of the signal. Secondly, the deep convolutional neural network is used to extract the hierarchical features of the ECG signal. Finally, the test is performed on the test data set, and the classifier is used for classification. The implementation includes the following steps:
[0065] Step S1, select data set
[0066] Due to the characteristic that the electrocardiogram signal is susceptible to interference, the present invention selects the electrocardiogram data set provided by the 2017 PhysioNet / CINC Challenge as the judge. 2017 PhysiNet / CINC challen...
Embodiment 2
[0102] as attached figure 1 Shown, a kind of electrocardiogram classification device based on wavelet transform and DCNN of the present invention comprises:
[0103] The data set sampling module selects the data set and samples the electrocardiogram data in the data set;
[0104] The data set preprocessing module uses wavelet transform to preprocess the ECG data in the data set to obtain ECG data test samples;
[0105] The deep convolution and feature extraction module uses the deep convolutional neural network DCNN to extract the hierarchical features of the ECG signal, and uses a larger convolution kernel than the extracted image features to expand the perception field of convolution and at the same time use one-dimensional convolution Accumulate kernel to extract features of ECG signal;
[0106] Classifier module: use the classifier to predict the ECG data test samples and output the classification results;
[0107] Evaluation indicator module: evaluate the classificatio...
PUM
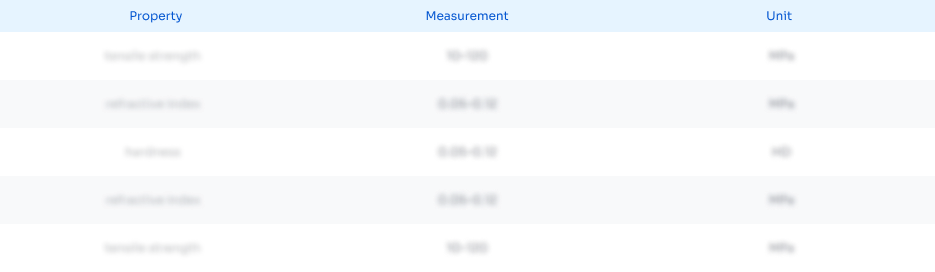
Abstract
Description
Claims
Application Information
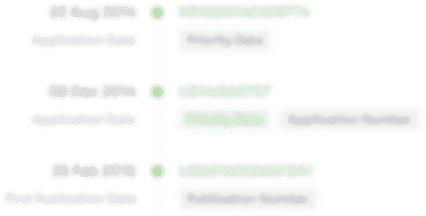
- R&D Engineer
- R&D Manager
- IP Professional
- Industry Leading Data Capabilities
- Powerful AI technology
- Patent DNA Extraction
Browse by: Latest US Patents, China's latest patents, Technical Efficacy Thesaurus, Application Domain, Technology Topic, Popular Technical Reports.
© 2024 PatSnap. All rights reserved.Legal|Privacy policy|Modern Slavery Act Transparency Statement|Sitemap|About US| Contact US: help@patsnap.com