Long-distance pipeline inspection method based on YOLOv3 pruning network and deep learning defogging model
A deep learning and network model technology, applied in the field of image processing, can solve the problems of weakening image feature extraction, poor image quality, and high labor costs, and achieve the effects of improving real-time performance, improving efficiency, and reducing labor costs
- Summary
- Abstract
- Description
- Claims
- Application Information
AI Technical Summary
Problems solved by technology
Method used
Image
Examples
Embodiment 1
[0063] Such as figure 1 As shown, the present invention is a long-distance pipeline inspection method based on the deep learning dehazing algorithm and the YOLOv3 channel pruning network. The AOD-Net model is used to dehaze the aerial images of UAVs in foggy scenes to obtain high-quality and clear images. The image is sent to the YOLOv3 channel to calculate the accelerated compression model after pruning, which is conducive to improving the detection accuracy and achieving real-time detection. The specific implementation steps are as follows:
[0064] S1: Construct and train the AOD-Net dehazing network model for image dehazing and haze removal, such as image 3 shown;
[0065] S101: The classical atmospheric light scattering model is transformed into formulas, and the physical model of atmospheric light scattering is:
[0066] I(x)=J(x)t(x)+A(1-t(x))
[0067] Among them, I(x) represents the haze image, J(x) is the scene radiation, which is the clear image to be restored, A...
PUM
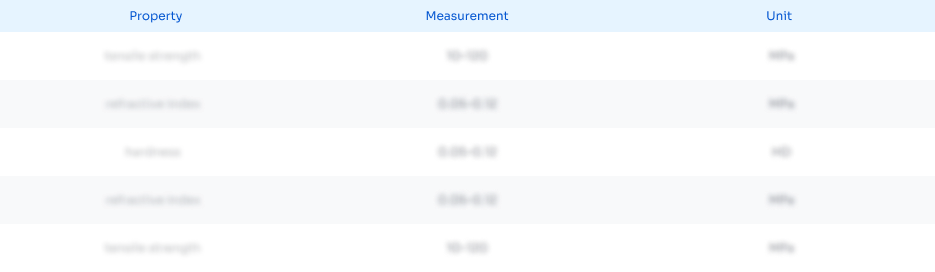
Abstract
Description
Claims
Application Information
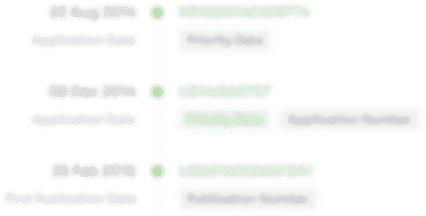
- R&D Engineer
- R&D Manager
- IP Professional
- Industry Leading Data Capabilities
- Powerful AI technology
- Patent DNA Extraction
Browse by: Latest US Patents, China's latest patents, Technical Efficacy Thesaurus, Application Domain, Technology Topic.
© 2024 PatSnap. All rights reserved.Legal|Privacy policy|Modern Slavery Act Transparency Statement|Sitemap