Intelligent power grid deep learning scheduling method considering schedulable electric vehicle fast/slow charging and discharging forms
An electric vehicle and smart grid technology, applied in electric vehicle charging technology, machine learning, computing, etc., can solve problems such as unconsidered adverse effects and the impact of grid scheduling plans
- Summary
- Abstract
- Description
- Claims
- Application Information
AI Technical Summary
Problems solved by technology
Method used
Image
Examples
Embodiment Construction
[0114] The present invention will be further described below in conjunction with specific examples.
[0115] In this paper, the smart grid dispatching system is mainly composed of two parts: the power supply end and the load end, such as figure 2 As shown, the power generation side includes V2G systems, thermal power units and wind farms, and the load side includes conventional loads, a large number of disordered electric vehicles and a large number of dispatchable electric vehicle charging loads. In order to reduce the start-up and stop costs of thermal power units, during the day-ahead scheduling process, the two largest conventional units in the thermal power units are always on, so the thermal power units are divided into thermal power unit I and thermal power unit II, and thermal power unit I is composed of the two largest conventional units Composition of conventional units, thermal power unit II is composed of other units. The present invention uses the 10-unit system ...
PUM
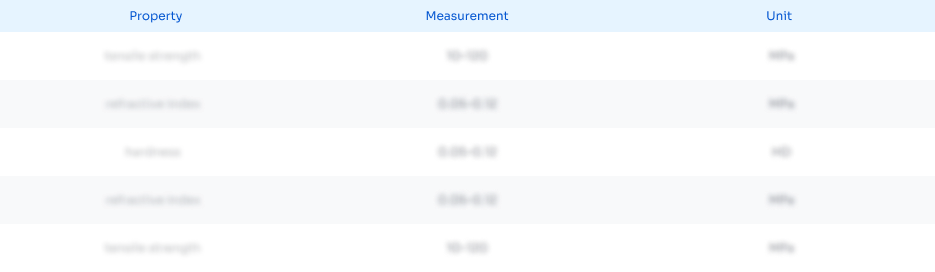
Abstract
Description
Claims
Application Information
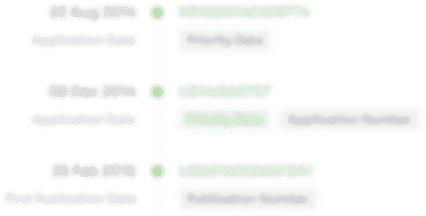
- R&D
- Intellectual Property
- Life Sciences
- Materials
- Tech Scout
- Unparalleled Data Quality
- Higher Quality Content
- 60% Fewer Hallucinations
Browse by: Latest US Patents, China's latest patents, Technical Efficacy Thesaurus, Application Domain, Technology Topic, Popular Technical Reports.
© 2025 PatSnap. All rights reserved.Legal|Privacy policy|Modern Slavery Act Transparency Statement|Sitemap|About US| Contact US: help@patsnap.com