Plasma protein marker, detection reagent or detection tool for diagnosing severe-to-critical symptom of new coronal virus pneumonia
A technology for turning critical illnesses and proteins, which is applied in biological testing, disease diagnosis, and microbial detection/testing, and can solve the problems of no biomarkers for the occurrence, development, and outcome of coronavirus disease
- Summary
- Abstract
- Description
- Claims
- Application Information
AI Technical Summary
Problems solved by technology
Method used
Image
Examples
Embodiment 1
[0034] Example 1: Mass Spectrometry Analysis of Plasma Samples from Patients with New Coronary Pneumonia
[0035] 1. Experimental materials
[0036] Plasma samples from patients with new coronary pneumonia, from a hospital, including whole blood collected from 7 critically ill patients (S1-S7, referred to as S), and whole blood collected from 5 dead patients at 4 time points during hospitalization (the last one The time points were taken before death, F1T1-F1T4, F2T1-F2T4, F3T1-F3T4, F4T1-F4T4, F5T1-F5T4, referred to as FT1-FT4), a total of 27 whole blood samples.
[0037] The clinical classification is as follows: severe, meeting any of the following: 1. shortness of breath, RR ≥ 30 times / min; 2. resting state, oxygen saturation ≤ 93%; 3. partial pressure of oxygen in arterial blood (Pa02) / Oxygen inhalation concentration (Fi02)≤300mg1mmHg=0.1Pa), high altitude (over 1000 meters above sea level) areas should correct Pa02 / Fi02 according to the following formula: Pa02 / F102×[at...
Embodiment 2
[0044] Example 2: Screening markers for critical illness to critical illness by machine learning
[0045] 1. Experimental materials
[0046] Mass spectrum data of 27 plasma samples (sample described in Example 1), Python 3.7 (https: / / www.anaconda.com / ), Scikit learn 0.22.1 (https: / / scikit-learn.org / stable / ) . The source code of this experiment is https: / / github.com / Ning-310 / POC-19.
[0047] 2. Experimental process
[0048] (1) To screen for differential proteins, to screen for the absolute value of the fold change (FC) of the proteins in the S group and the FT1-FT4 group to be greater than 0.8, and the two-tailed unpaired Welch T test to be less than 0.01 (|log2(FC)|>0.8, unpaired two-sided Welch's t-test, P<0.01).
[0049] (2) Randomly select no more than 5 proteins from the differential proteins to form a potential optimal marker combination (OBC). The initial weight value of each protein is set to 1, and 1000 OBC candidates are set.
[0050] (3) For each candidate OBC,...
Embodiment 3
[0058] Example 3: Validation of OBC Predictor Accuracy
[0059] 1. Experimental materials
[0060] Mass spectrum data of 27 plasma samples (samples described in Example 1), Python 3.7 (https: / / www.anaconda.com / ).
[0061] 2. Experimental process
[0062] (1) The identified proteins in OBC are divided into true positive (TruePositive, TP), true negative (TrueNegative, TN), false positive (False Positive, FP) and false negative (FalseNegative, FalseNegative, FN) ratio for chaos logic analysis.
[0063] (2) Draw the chaotic matrix through Scikit learn 0.22.1 software. Principal component analysis (PCA) was used to test the degree of separation of the two sets of data predicted by OBC.
[0064] The result is as image 3 As shown, the OBC combination predicts the true positive rate of 88% and the false positive rate of 12%.
PUM
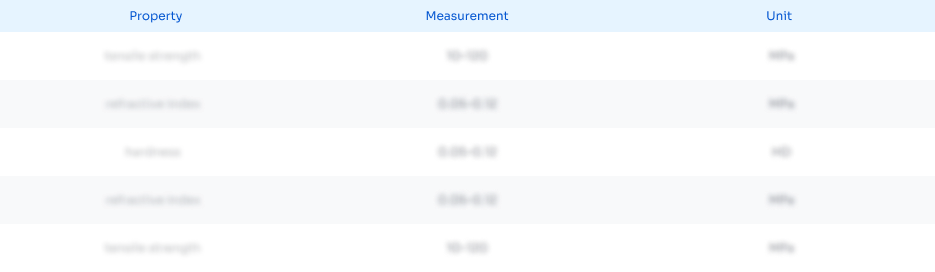
Abstract
Description
Claims
Application Information
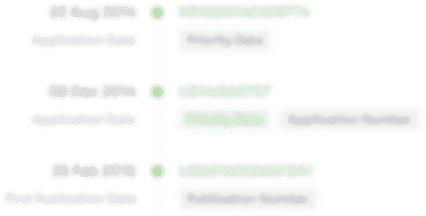
- R&D Engineer
- R&D Manager
- IP Professional
- Industry Leading Data Capabilities
- Powerful AI technology
- Patent DNA Extraction
Browse by: Latest US Patents, China's latest patents, Technical Efficacy Thesaurus, Application Domain, Technology Topic.
© 2024 PatSnap. All rights reserved.Legal|Privacy policy|Modern Slavery Act Transparency Statement|Sitemap