Power load probability prediction system and method for multi-core intelligent meter
A probabilistic forecasting and power load technology, applied in forecasting, neural learning methods, data processing applications, etc., to achieve the effects of preventing overfitting, improving robustness, and fast training speed
- Summary
- Abstract
- Description
- Claims
- Application Information
AI Technical Summary
Problems solved by technology
Method used
Image
Examples
Embodiment Construction
[0049] refer to figure 1 As shown, a power load probabilistic forecasting system for multi-core smart meters, including convolutional neural network CNN, recurrent neural network GRU and mixed density network. The convolutional neural network CNN includes a convolutional layer and a pooling layer; the convolutional neural network CNN uses ReLU as the activation function; the pooling layer can discard 20% of the data and output it to the flatten layer to prevent overfitting during the training process; The convolutional neural network CNN and the cyclic neural network GRU are connected through a flatten layer. The flatten layer is used to reduce the dimensionality of the spatial features, and then form a feature vector with the historical load data, which is output to the cyclic neural network GRU.
[0050] The convolutional neural network is used to extract spatial features from historical environmental factor data, and construct spatial features and corresponding historical l...
PUM
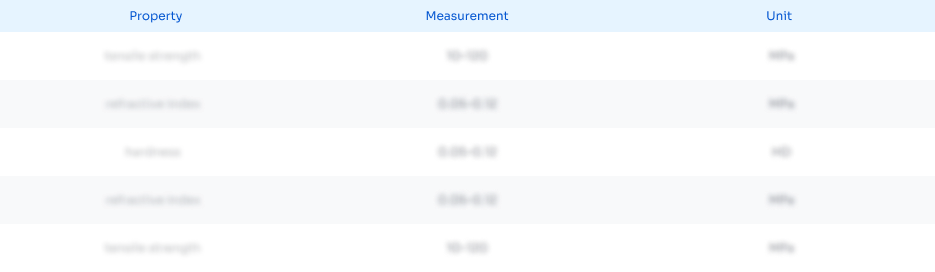
Abstract
Description
Claims
Application Information
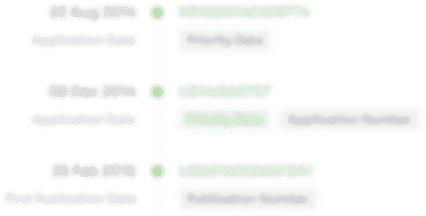
- R&D Engineer
- R&D Manager
- IP Professional
- Industry Leading Data Capabilities
- Powerful AI technology
- Patent DNA Extraction
Browse by: Latest US Patents, China's latest patents, Technical Efficacy Thesaurus, Application Domain, Technology Topic.
© 2024 PatSnap. All rights reserved.Legal|Privacy policy|Modern Slavery Act Transparency Statement|Sitemap