Lithium battery life prediction method based on LSTM network and transfer learning
A technology of life prediction and transfer learning, which is applied in the field of lithium-ion batteries, can solve problems such as small error value, gradient explosion, and gradient disappearance, so as to improve efficiency, reduce training time, and avoid gradient disappearance and explosion.
- Summary
- Abstract
- Description
- Claims
- Application Information
AI Technical Summary
Problems solved by technology
Method used
Image
Examples
Embodiment
[0075] The experimental data of the present invention comes from the laboratory lithium ion battery life test experiment. The rated capacity of the battery is 1350mAh, and the number of battery cycles and the battery capacity of the lithium-ion battery for each cycle are extracted and stored through experiments. The charge and discharge data group contains time, charge and discharge voltage, and charge and discharge current data structure, including the number of battery cycles and battery capacity. The lithium-ion battery is first charged with a constant current of 0.675A until the battery voltage reaches 4.2V, and maintained at 4.2V until the charging current drops below 0.05A; it is discharged at a constant current of 0.55A until the battery voltage drops to 2.7V. In the experiment conducted by CALCE, the capacity of the lithium-ion battery reaches 1080mAh, which is about 80% of the rated capacity, and the experiment is over, so the end-of-life threshold of the battery her...
PUM
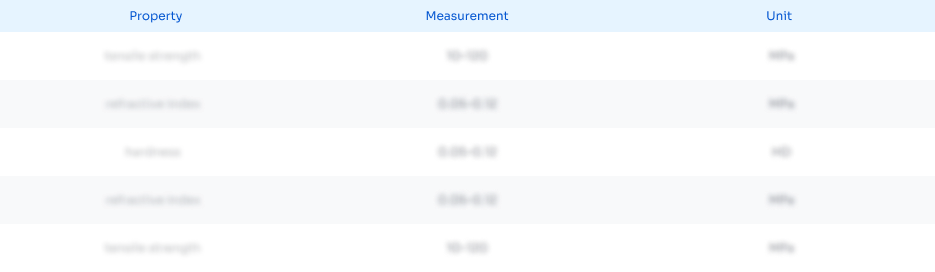
Abstract
Description
Claims
Application Information
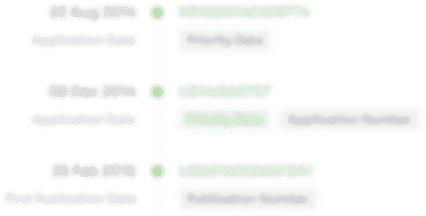
- Generate Ideas
- Intellectual Property
- Life Sciences
- Materials
- Tech Scout
- Unparalleled Data Quality
- Higher Quality Content
- 60% Fewer Hallucinations
Browse by: Latest US Patents, China's latest patents, Technical Efficacy Thesaurus, Application Domain, Technology Topic, Popular Technical Reports.
© 2025 PatSnap. All rights reserved.Legal|Privacy policy|Modern Slavery Act Transparency Statement|Sitemap|About US| Contact US: help@patsnap.com