CT image black band artifact elimination method and system based on deep learning
A CT image, deep learning technology, applied in image enhancement, image analysis, image data processing and other directions, can solve the problems of CT image CT value is too large, complex image segmentation, image detail changes and other problems
- Summary
- Abstract
- Description
- Claims
- Application Information
AI Technical Summary
Problems solved by technology
Method used
Image
Examples
Embodiment 1
[0067] Such as figure 1 As shown, the CT image black band artifact elimination method based on deep learning of the present embodiment comprises the following steps:
[0068] S1. CT image data acquisition
[0069] Acquisition of CT images with black band artifacts and non-matching CT images without black band artifacts;
[0070] S2, artifact removal and noise addition
[0071] The two-pass algorithm is used to remove artifacts from CT images with black band artifacts, and the artifact-removed CT images with CT value shifts are obtained; although the artifact-removed CT images with CT value shifts obtained by the two-pass algorithm are not Ideal, but the information at the black belt artifact can be restored and the image details can be completely preserved, which can make up for the shortcomings of Cycle-GAN.
[0072] Gaussian noise is added to the CT image without black band artifacts to obtain a noisy CT image;
[0073] S3, data processing
[0074] The CT images with bl...
Embodiment 2
[0130] The difference between the deep learning-based CT image black band artifact elimination method and system of this embodiment and embodiment 1 is:
[0131] The algorithm for artifact elimination was replaced by a two-pass algorithm with a multi-channel cone beam artifact reduction algorithm (see Chulhee, Han, Jongduk.Multi-pass approach to reduce cone-beam artifacts in acircular orbit cone-beam CT system.[J ]. Optics Express, 2019) or an adaptive two-pass cone-beam artifact correction algorithm based on FOV-preserving dual-source geometry (see Forthmann P, Grass M, Proksa R. Adaptive two-pass cone-beam artifact correction using a FOV-preserving two -sourcegeometry:A simulation study[J].Medical Physics,2009,36(10):4440–4450), to meet the needs of different applications.
[0132] For other steps of the method and system architecture, reference may be made to Embodiment 1.
Embodiment 3
[0134] The difference between the deep learning-based CT image black band artifact elimination method and system of this embodiment and embodiment 1 is:
[0135] The added noise is replaced by Gaussian noise to Poisson noise to meet the needs of different applications.
[0136] For other steps of the method and system architecture, reference may be made to Embodiment 1.
PUM
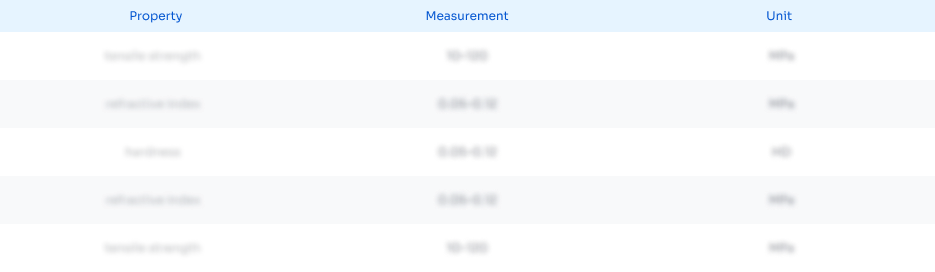
Abstract
Description
Claims
Application Information
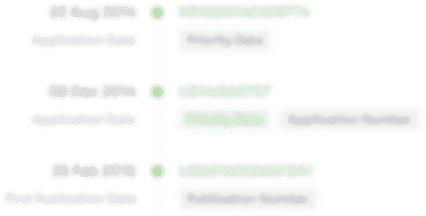
- R&D Engineer
- R&D Manager
- IP Professional
- Industry Leading Data Capabilities
- Powerful AI technology
- Patent DNA Extraction
Browse by: Latest US Patents, China's latest patents, Technical Efficacy Thesaurus, Application Domain, Technology Topic, Popular Technical Reports.
© 2024 PatSnap. All rights reserved.Legal|Privacy policy|Modern Slavery Act Transparency Statement|Sitemap|About US| Contact US: help@patsnap.com