Fault positioning method for mining traveling wave time-frequency domain characteristics by using deep learning
A technology of deep learning and fault location, which is applied in the field of power system, can solve the problems of large positioning error, positioning failure, and low reliability of traveling wave positioning, and achieve the effect of avoiding calculation errors and improving reliability and accuracy
- Summary
- Abstract
- Description
- Claims
- Application Information
AI Technical Summary
Problems solved by technology
Method used
Image
Examples
Embodiment Construction
[0034] The following will clearly and completely describe the technical solutions in the embodiments of the present invention with reference to the accompanying drawings in the embodiments of the present invention. Obviously, the described embodiments are only some, not all, embodiments of the present invention. Based on the embodiments of the present invention, all other embodiments obtained by persons of ordinary skill in the art without making creative efforts belong to the protection scope of the present invention.
[0035] The embodiment of the present invention discloses a fault location method using deep learning to mine time-frequency domain features of traveling waves, such as figure 1 Shown is the flow chart of the inventive method, comprises the following steps:
[0036] Step 1: Obtain the fault traveling wave line mode components under various fault conditions.
[0037] Specifically, the three-phase voltage traveling wave data detected at various positions on the ...
PUM
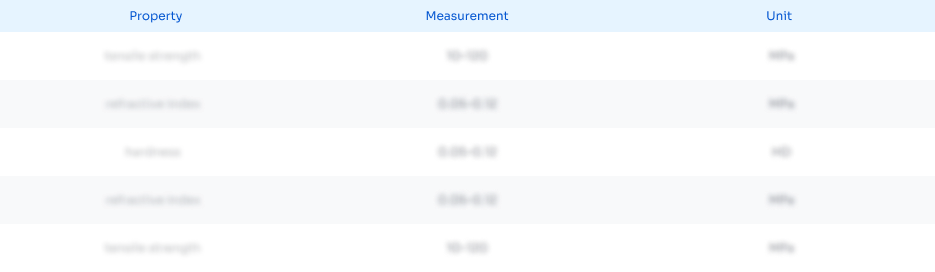
Abstract
Description
Claims
Application Information
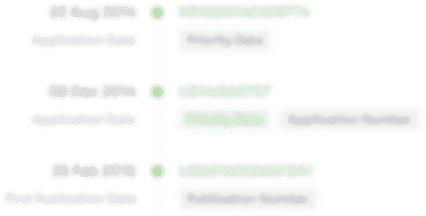
- Generate Ideas
- Intellectual Property
- Life Sciences
- Materials
- Tech Scout
- Unparalleled Data Quality
- Higher Quality Content
- 60% Fewer Hallucinations
Browse by: Latest US Patents, China's latest patents, Technical Efficacy Thesaurus, Application Domain, Technology Topic, Popular Technical Reports.
© 2025 PatSnap. All rights reserved.Legal|Privacy policy|Modern Slavery Act Transparency Statement|Sitemap|About US| Contact US: help@patsnap.com