Algorithm for automatically identifying non-perfusion area of fundus fluorescence angiography image and recommending laser photocoagulation area
A technology of contrast-enhanced images and automatic recognition, which is applied in the field of intelligent medical care, can solve problems that have not been reported, and achieve the effect of improving disease analysis and diagnosis ability
- Summary
- Abstract
- Description
- Claims
- Application Information
AI Technical Summary
Problems solved by technology
Method used
Image
Examples
Embodiment 1
[0042] Construction of Neural Network Model
[0043] Three standardized Unet convolutional neural network models were constructed based on the Keras deep learning framework with TensorFlow as the backend, which were used to identify the optic disc area, macular area, and non-perfusion area. Build various layers of the network model through the layers module in the keras framework. The method of building the convolution layer is layers.Conv2D, the method of building the pooling layer is layers.MaxPooling2D, and the method of building the transposed convolution layer is layers.Upsampling2D. The splicing of high-level features and low-level features uses the layers.concatenate method.
[0044] Network model structure description: The network model has a total of 34 layers, including 4 parts: input layer, downsampling layer, upsampling layer, and output layer.
[0045] The input is all contrast images with a size of 768*768.
[0046] The downsampling layer consists of the first ...
Embodiment 2
[0050] The optimization of the network model includes the following steps:
[0051] Step 1: A professional physician collects a 55-degree fundus angiography image of the posterior pole of the fundus, and the collection device is Heidelberg SPECTRALIS HRA+OCT.
[0052] Step 2: Image desensitization preprocessing: Use the cv2.findContours method under the opencv-python module to extract all the contours of the fundus area, among which the retinal contour has the largest contour area, and remove the patient information on the image and set the background area to 0.
[0053] For desensitization pretreatment results, see figure 1 ,in figure 1 -a is the extraction result of the fundus area contour, and the dotted line range is the extraction result of the fundus area; figure 1 -b is the output result of desensitization, which removes patient information and other content; figure 1 -c is the final result after preprocessing.
[0054] Step 3: Use the Contrast Limited Adaptive Hist...
Embodiment 3
[0062] Recognition and Segmentation of Fundus Imaging Based on Multi-Stream Convolutional Neural Network Model
[0063]Load the collected patient's fundus contrast image into the trained network model to obtain the probability that each pixel of the contrast image belongs to each category. The fundus contrast images are identified and segmented as follows.
[0064] 1. Positioning of the center of the disc:
[0065] (1) Select the maximum value p_max and the minimum value p_min of all pixel prediction probabilities; (2) determine the dynamic threshold for separating the optic disc and the background, p_thresh=(1-k)*p_min+k*p_max, where p_tresh represents the separation of the optic disc and the background The threshold value of k is 0.8; (3) Select the pixel points greater than the threshold value as the candidate area of the optic disc, such as Figure 4 -shown in -a; (4) open operation to the disc region; (5) traverse each connected region S in the candidate region, and j...
PUM
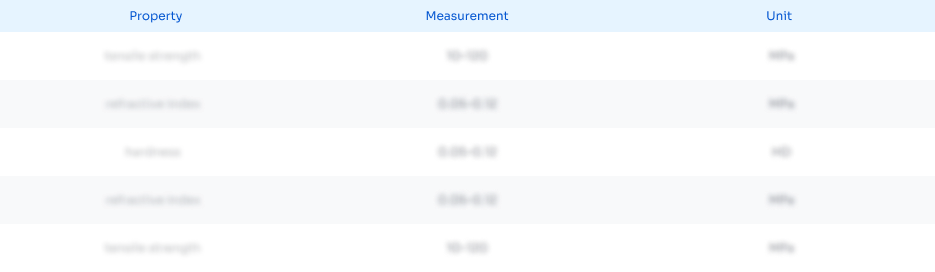
Abstract
Description
Claims
Application Information
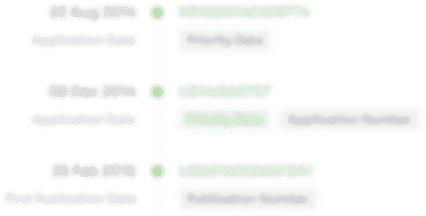
- R&D Engineer
- R&D Manager
- IP Professional
- Industry Leading Data Capabilities
- Powerful AI technology
- Patent DNA Extraction
Browse by: Latest US Patents, China's latest patents, Technical Efficacy Thesaurus, Application Domain, Technology Topic, Popular Technical Reports.
© 2024 PatSnap. All rights reserved.Legal|Privacy policy|Modern Slavery Act Transparency Statement|Sitemap|About US| Contact US: help@patsnap.com