Weld defect identification and positioning method and system based on deep learning network
A deep learning network, defect recognition technology, applied in character and pattern recognition, biological neural network model, image data processing and other directions, can solve the problems of low accuracy, insufficient recognition and positioning accuracy, low detection efficiency, etc., to improve the accuracy rate and speed, improve engineering application value, and improve the effect of identification and positioning accuracy
- Summary
- Abstract
- Description
- Claims
- Application Information
AI Technical Summary
Problems solved by technology
Method used
Image
Examples
Embodiment 1
[0082] Such as figure 1 As shown, this embodiment provides a method for identifying and locating weld defects based on a deep learning network. The method includes the following steps:
[0083] S1: Obtain radiographic images of weld defects containing pores, slag inclusions, cracks, incomplete fusion and incomplete penetration defects, and use some of the images as training data sets, and the rest as test data sets.
[0084] S2: Perform normalized preprocessing on the images in the test data set or the training data set, after the preprocessing, a block with uniform resolution of the weld defect image is obtained;
[0085] The image preprocessing method is: cut the collected original image according to the specification of 320×320 pixels, and generate the image as an input block. For the original image whose width and height are not an integral multiple of 320×320, the overlap in the graphics is partially reserved. The cutting is done in the way of regions, so that all the cu...
Embodiment 2
[0146] This embodiment provides a simulation experiment of the method for identifying and locating weld defects based on a deep learning network as in Embodiment 1 (in other embodiments, the simulation experiment may not be performed, and other experimental schemes may also be used to conduct experiments to determine the method. The effect of the network model and its related parameters on the weld recognition and localization performance of the method).
[0147] (1) Experimental conditions
[0148] In this embodiment, the detection experiment uses the operating system Windows10, CPUi7-8700k, GPU is 1080ti, memory is 16GB, and the deep learning framework is tensorflow.
[0149] Initialization parameter settings for network training: maximum iteration 50,000 times, learning rate 0.001, batch_size setting 32, weight attenuation coefficient 0.0005, impulse constant 0.9, according to the trend of loss decline, adjust the learning rate and batch_size values appropriately until th...
Embodiment 3
[0183] This embodiment provides a system for identifying and locating weld defects based on a deep learning network. The system uses the method for identifying and locating weld defects based on a deep learning network as in Embodiment 1 to complete the identification of weld defects in radiographic images of welds. and positioning, and give prediction results; the system includes: image acquisition module 1, image preprocessing module 2, and identification and positioning network module 3.
[0184] The image acquisition module 1 is used to acquire radiographic images of weld defects containing pores, slag inclusions, cracks, incomplete fusion and incomplete penetration defects, and use the images as a training set or test set, and complete the alignment based on the images in the training set or test set System training, or complete the task of identifying and locating weld defects in images.
[0185] The image preprocessing module 2 is used to perform normalized preprocessin...
PUM
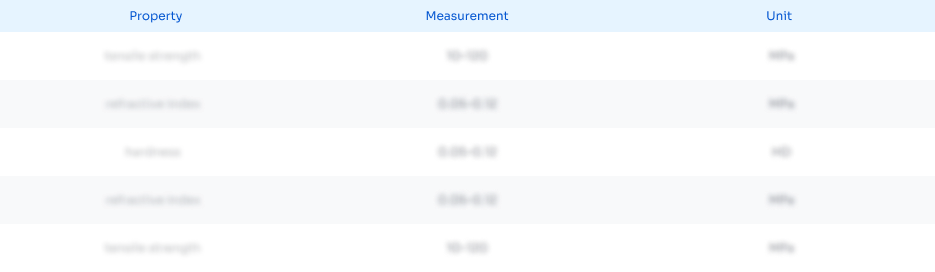
Abstract
Description
Claims
Application Information
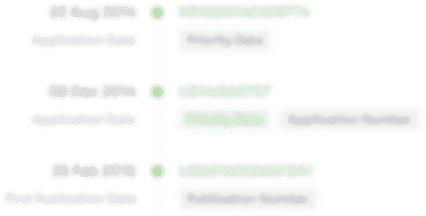
- Generate Ideas
- Intellectual Property
- Life Sciences
- Materials
- Tech Scout
- Unparalleled Data Quality
- Higher Quality Content
- 60% Fewer Hallucinations
Browse by: Latest US Patents, China's latest patents, Technical Efficacy Thesaurus, Application Domain, Technology Topic, Popular Technical Reports.
© 2025 PatSnap. All rights reserved.Legal|Privacy policy|Modern Slavery Act Transparency Statement|Sitemap|About US| Contact US: help@patsnap.com