Long-time-sequence traffic flow prediction method based on graph convolution-Informer model
A technology of traffic flow and prediction method, which is applied in the traffic control system of road vehicles, forecasting, traffic control system, etc., which can solve the insensitivity of traffic flow peaks, lack of analysis of high-dimensional time series information, and insensitivity to periodic distribution changes of traffic flow and other issues to achieve the effect of improving efficiency
- Summary
- Abstract
- Description
- Claims
- Application Information
AI Technical Summary
Problems solved by technology
Method used
Image
Examples
Embodiment Construction
[0049] like figure 1 and figure 2 As shown, a kind of long-sequence traffic flow prediction method based on graph convolution-Informer model of the present invention comprises the following steps:
[0050] Step 1: Build a data set: Collect the speed information of all passing vehicles at the expressway station and the provincial trunk road interchange station per unit time, and establish the traffic flow time series information data set X after data preprocessing;
[0051] Step 2: Establish a topology map of the site network structure: establish a topology map of the site network structure based on the relative geographical location information of the expressway site and the intermodulation site of the provincial trunk road, specifically to establish the adjacency matrix A of all sites, and calculate the symmetry of the spatial information of the site Normalized Laplace matrix
[0052] Step 3: Build a graph convolutional neural network model: construct a two-layer graph c...
PUM
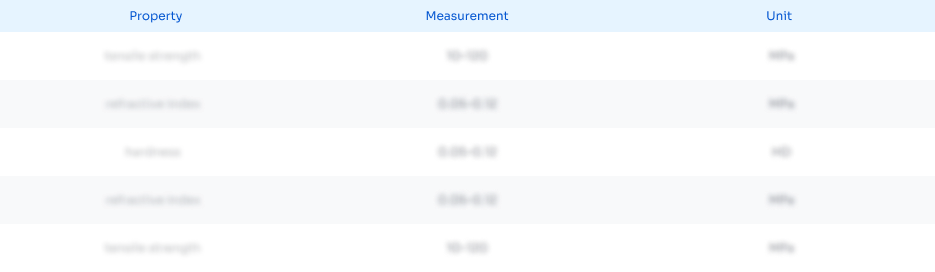
Abstract
Description
Claims
Application Information
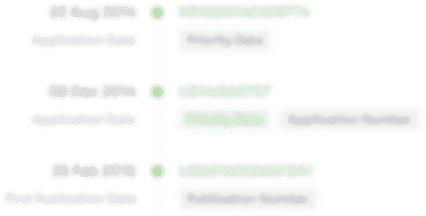
- R&D Engineer
- R&D Manager
- IP Professional
- Industry Leading Data Capabilities
- Powerful AI technology
- Patent DNA Extraction
Browse by: Latest US Patents, China's latest patents, Technical Efficacy Thesaurus, Application Domain, Technology Topic, Popular Technical Reports.
© 2024 PatSnap. All rights reserved.Legal|Privacy policy|Modern Slavery Act Transparency Statement|Sitemap|About US| Contact US: help@patsnap.com