Skin lesion segmentation method based on convolution attention model
An attention model, a technology for skin lesions, applied in neural learning methods, biological neural network models, image analysis, etc., can solve the problems of incomplete lesion area, large amount of parameters, imperfect feature extraction, etc., to improve feature representation, The effect of improving performance
- Summary
- Abstract
- Description
- Claims
- Application Information
AI Technical Summary
Problems solved by technology
Method used
Image
Examples
Embodiment 1
[0043] Such as Figure 1-5 As shown, the present invention provides a kind of skin lesion segmentation method based on convolutional attention model, and this segmentation method specifically follows the steps:
[0044] Step 1: Select ISIC-2017 dataset and PH2 dataset:
[0045] The ISIC-2017 dataset contains 8-bit RGB dermoscopy images ranging in size from 540×722-4499×6748 pixels. The dataset provides 2000 training images, a separate dataset of 150 images for validation, and a separate dataset for A separate dataset of 600 images was tested. All dermoscopy images in the dataset were labeled as benign moles, melanomas, or seborrheic keratoses. The PH2 dataset contained 200 images, of which 160 were moles. are common moles and atypical moles, and the remaining 40 images are melanomas. The images in the PH2 dataset are 8-bit RGB images with a fixed size of 768×560 pixels, collected under the same conditions with a magnification of 20 times;
[0046] Step 2: Data preprocessing:...
PUM
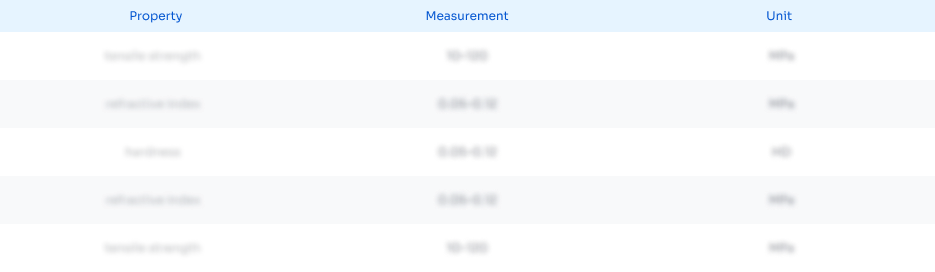
Abstract
Description
Claims
Application Information
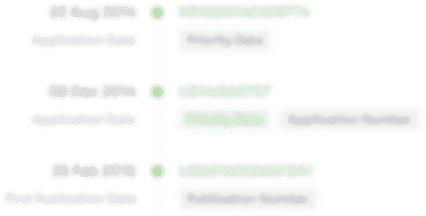
- R&D
- Intellectual Property
- Life Sciences
- Materials
- Tech Scout
- Unparalleled Data Quality
- Higher Quality Content
- 60% Fewer Hallucinations
Browse by: Latest US Patents, China's latest patents, Technical Efficacy Thesaurus, Application Domain, Technology Topic, Popular Technical Reports.
© 2025 PatSnap. All rights reserved.Legal|Privacy policy|Modern Slavery Act Transparency Statement|Sitemap|About US| Contact US: help@patsnap.com