Method and system for multidimensional target prediction of disease-associated non-coding RNA regulatory axis
A prediction method and technology of regulating axis, which can be applied in the fields of biostatistics, bioinformatics, instruments, etc., can solve the problem of inability to identify functional modules of lncRNA-miRNA-mRNA regulatory axis, and achieve the effect of improving reliability.
- Summary
- Abstract
- Description
- Claims
- Application Information
AI Technical Summary
Problems solved by technology
Method used
Image
Examples
Embodiment 1
[0042] This Example 1 provides a newly optimized xgboost algorithm and multi-organized data integration analysis in an analytical application in endogenous competition non-encoded RNA regulatory networks of complex diseases, specifically divided into the following steps:
[0043] Step 1: Data acquisition and pretreatment. By pretreatment of corresponding bioinformatics analysis of at least three dimensions (e.g., genomic, transcription group and methylation data), the weight gene co-expression network analysis screening out of the disease group and the control group Difference expression gene and co-expression gene. The resulting results of the screening were enriched, and the protein-protein interaction (PPI) network was constructed, and the composition of the hub gene was finally determined.
[0044] In this Example 1, a lot of difference expression genes were obtained when differential expression analysis, and these genes were difficult to find the relationship between these ge...
Embodiment 2
[0060] In this Example 2, a disease-associated non-encoded RNA regulating axis multidimensional target prediction system, including:
[0061] Screening modules for screening the differential expression genes and co-expression gene modules between disease groups and control groups using multi-dimensional component data, and perform enrichment analysis;
[0062] The module is determined, and the constructed protein-protein interaction network is used to treat the screened differentially expression gene and co-expression gene to determine the composition of the hub gene;
[0063] Get the module for acquiring key protein coding markers in the determined hub gene;
[0064] The prediction module is used to extract a non-encoded RNA regulating axis network including the key protein coding marker using the constructed competitive endogenous RNA network.
[0065] In this Example 2, a multi-dimensional target prediction method of complex disease-related non-encoded RNA regulatory shaft is r...
Embodiment 3
[0081] Such as figure 1 As shown in the present embodiment, a multi-dimensional target-based non-encoded RNA regulating axis prediction method based on an optimized machine learning XGBOOST algorithm is provided, and the implementation of the following three steps:
[0082] Step 1: Screening the potential difference biomarkers through multiple groups of student information databases;
[0083] The specific steps include: Download disease-related genome, transcription group and methylation data via the GEO database. For example, Download transcription group data (GSE154377, GSE150621), expression profile data (GSE87295) and methylation data (GSE88929), and gene expression synthesis (GSE 112168) (GSE 112168) .
[0084] The above data is pretreated to retain appropriate data of differentially expressing genes, methylated genes, and miRNA. According to the T-SNE algorithm and the relevant matrix analysis, the sample of the Diabetic Group and the control group in the control group was r...
PUM
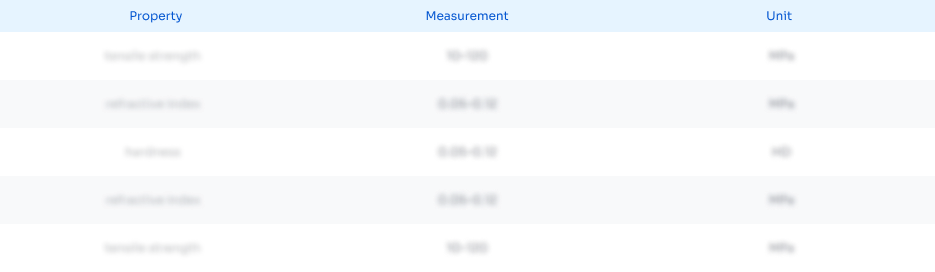
Abstract
Description
Claims
Application Information
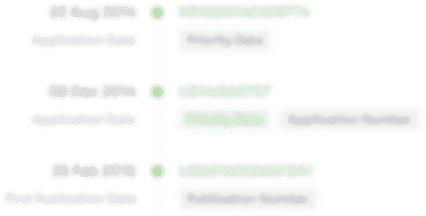
- R&D Engineer
- R&D Manager
- IP Professional
- Industry Leading Data Capabilities
- Powerful AI technology
- Patent DNA Extraction
Browse by: Latest US Patents, China's latest patents, Technical Efficacy Thesaurus, Application Domain, Technology Topic, Popular Technical Reports.
© 2024 PatSnap. All rights reserved.Legal|Privacy policy|Modern Slavery Act Transparency Statement|Sitemap|About US| Contact US: help@patsnap.com