Knowledge representation learning method based on graph convolutional network and translation model
A technology of translation model and knowledge representation, which is applied in the field of knowledge representation learning to achieve the effect of improving performance
- Summary
- Abstract
- Description
- Claims
- Application Information
AI Technical Summary
Problems solved by technology
Method used
Image
Examples
Embodiment Construction
[0029] The knowledge base of the present invention adopts Comparative Toxicogenomics Database (Comparative Toxicogenomics Database, CTD), and CTD is a knowledge base that includes knowledge such as the relationship between drugs and genes, the relationship between drugs and diseases, and the relationship between genes and diseases. The experiment uses the CTD knowledge base to obtain the relationship between disease and drug entities in large-scale unlabeled corpus, focusing on the study of drug-induced disease relationship.
[0030] Attached below figure 1 And technical scheme, further describe the concrete steps of the present invention:
[0031] Step 1: Use the text mining tool PubTator (Wei C H, Kao H Y, Lu Z. PubTator: aweb-based text mining tool for assisting biocuration [J]. Nucleic acids research, 2013, 41 (W1): W518-W522.) Publish all drug entities and disease entities and their corresponding MeSH IDs in the PubMed abstract; Guided by the Comparative Toxicogenomics D...
PUM
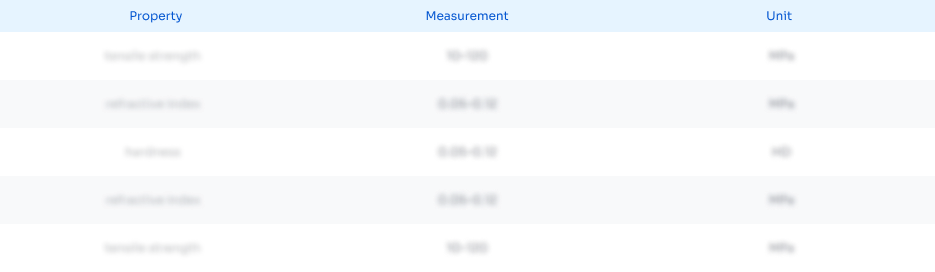
Abstract
Description
Claims
Application Information
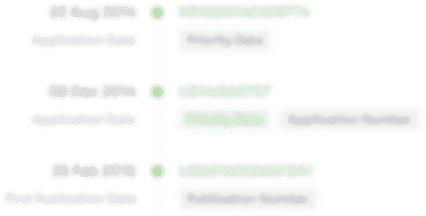
- R&D
- Intellectual Property
- Life Sciences
- Materials
- Tech Scout
- Unparalleled Data Quality
- Higher Quality Content
- 60% Fewer Hallucinations
Browse by: Latest US Patents, China's latest patents, Technical Efficacy Thesaurus, Application Domain, Technology Topic, Popular Technical Reports.
© 2025 PatSnap. All rights reserved.Legal|Privacy policy|Modern Slavery Act Transparency Statement|Sitemap|About US| Contact US: help@patsnap.com