AGV real-time path planning method based on optimal control and width learning
A technology of real-time path planning and optimal control, applied in the direction of non-electric variable control, control/adjustment system, two-dimensional position/channel control, etc., can solve the slow training process, complex adjustment of deep neural network parameters, and low time-consuming and other problems, to achieve the effect of long drawing time, high environmental requirements and low time consumption
- Summary
- Abstract
- Description
- Claims
- Application Information
AI Technical Summary
Problems solved by technology
Method used
Image
Examples
Embodiment 1
[0067] The path planning problem of AGV can be regarded as a trajectory planning problem in which the starting point of AGV is not fixed, but the end point (that is, the coordinate point of the delivery task) is fixed. The traditional trajectory method can be divided into: path search + trajectory optimization, but the traditional path search The method is often based on a grid map, and the searched route does not necessarily conform to the vehicle dynamics constraints (the vehicle cannot make a lateral movement). Therefore, it brings uncertainty in the optimization time and optimization quality to the later trajectory optimization. Therefore, in actual implementation, in order to ensure the effectiveness of path planning results, it is very necessary to consider the dynamic model of AGV as a constraint in the planning at the beginning stage. The trajectory planning problem with a fixed end point is solved in the forward direction by the optimal control method for solving the t...
Embodiment 2
[0121] In this embodiment, in addition to the training described in Embodiment 1 for the width learning network, since the magnitude of the data set is not within an order of magnitude, the training data set is normalized before being used for the width learning network training Processing, using methods including but not limited to max-min normalization, Z-score normalization and functional transformation.
[0122] The optimal state S and control rate C in the training data set with a certain position as the target state are input into the width learning network after training (for example, starting as ), the output of the width learning network needs to be inversely normalized, and finally used as a control rate that conforms to the physical meaning.
Embodiment 3
[0124]In this embodiment, on the basis of Embodiment 1 and Embodiment 2, focus on the discussion of AGV path planning that expands the initial point to any point and expands the destination to different end states. Under different starting points, the "optimal state-control rate" pair (s, c) trains a width learning network alone, and by combining the pseudo-inverse matrix solution of the incremental method, it can quickly learn the optimal state to the most optimal state. The weight matrix W of the optimal control mapping relationship can solve the optimal control problem when the starting point changes, and extend the initial point in the online optimal control to any point in the set area.
[0125] Among them, the number of feature nodes N in each group of the width learning network, the number of enhanced nodes M, and the number of newly added enhanced nodes can be selected according to the trade-off between computing power and prediction accuracy in specific scenarios.
[...
PUM
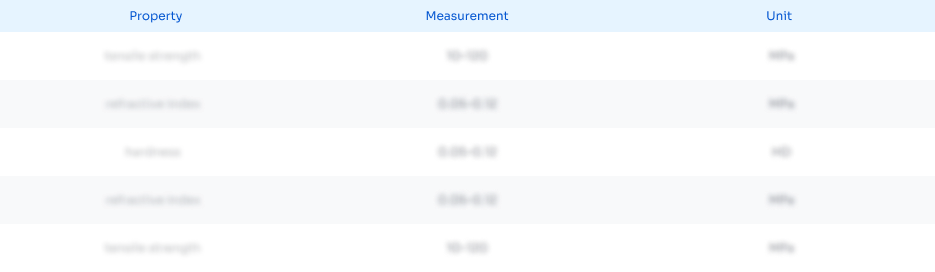
Abstract
Description
Claims
Application Information
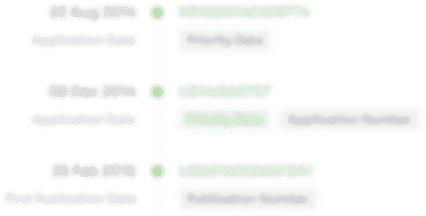
- R&D
- Intellectual Property
- Life Sciences
- Materials
- Tech Scout
- Unparalleled Data Quality
- Higher Quality Content
- 60% Fewer Hallucinations
Browse by: Latest US Patents, China's latest patents, Technical Efficacy Thesaurus, Application Domain, Technology Topic, Popular Technical Reports.
© 2025 PatSnap. All rights reserved.Legal|Privacy policy|Modern Slavery Act Transparency Statement|Sitemap|About US| Contact US: help@patsnap.com