Automobile sheet metal part surface defect detection method based on deep transfer learning
A technology of transfer learning and defect detection, which is applied in neural learning methods, computer components, image data processing, etc., can solve problems such as low fault tolerance rate, over-fitting, and affecting detection efficiency, so as to reduce the incidence of over-fitting, The effect of improving feature fitting ability and improving detection efficiency
- Summary
- Abstract
- Description
- Claims
- Application Information
AI Technical Summary
Problems solved by technology
Method used
Image
Examples
Embodiment Construction
[0054] The following will clearly and completely describe the technical solutions in the embodiments of the present invention with reference to the accompanying drawings in the embodiments of the present invention. Obviously, the described embodiments are only some, not all, embodiments of the present invention. Based on the embodiments of the present invention, all other embodiments obtained by persons of ordinary skill in the art without making creative efforts belong to the protection scope of the present invention.
[0055] see Figure 1-2 , the present invention provides a technical solution: a method for detecting surface defects of automobile sheet metal based on deep transfer learning, comprising the following steps:
[0056] S1: Tomographically scan sheet metal parts with an infrared thermal imager. For a product, hundreds of sliced images can be obtained in one scan. Experienced inspectors mark the collected images according to whether there are defects in the slic...
PUM
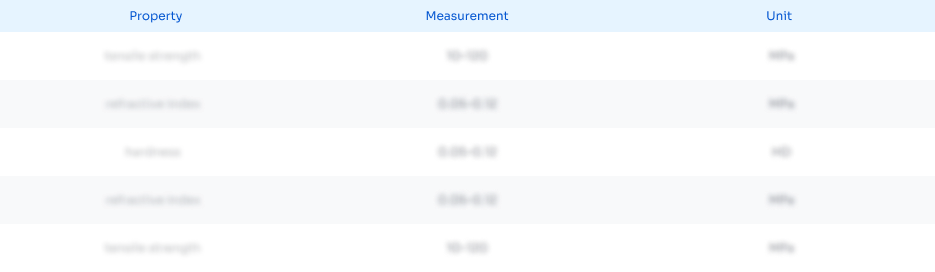
Abstract
Description
Claims
Application Information
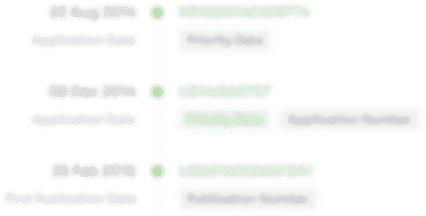
- R&D
- Intellectual Property
- Life Sciences
- Materials
- Tech Scout
- Unparalleled Data Quality
- Higher Quality Content
- 60% Fewer Hallucinations
Browse by: Latest US Patents, China's latest patents, Technical Efficacy Thesaurus, Application Domain, Technology Topic, Popular Technical Reports.
© 2025 PatSnap. All rights reserved.Legal|Privacy policy|Modern Slavery Act Transparency Statement|Sitemap|About US| Contact US: help@patsnap.com