Multi-supervision face in-vivo detection method fusing multi-scale features
A multi-scale feature, live detection technology, applied in deception detection, neural learning methods, biometric recognition and other directions, can solve the problem of increasing the time complexity of detecting living bodies, and achieve improved performance and generalization ability, good robustness, The effect of high detection accuracy
- Summary
- Abstract
- Description
- Claims
- Application Information
AI Technical Summary
Problems solved by technology
Method used
Image
Examples
Embodiment 1
[0043] refer to Figures 1 to 3 , which is an embodiment of the present invention, provides a multi-supervised face liveness detection method fused with multi-scale features, including:
[0044] S1: Collect an image dataset and preprocess the dataset.
[0045] It should be noted that three mainstream public image datasets, OULU-NPU, CASIA-MFSD and Replay-Attack, are used;
[0046] The performance included in the OULU-NPU dataset:
[0047] Evaluate the generalization ability of the model under different lighting and backgrounds;
[0048] Evaluate the generalization ability of the model under different attack methods;
[0049] Explore the impact of different shooting equipment on model performance;
[0050] Evaluate the general capabilities of the model under different scenarios, attack methods, and shooting equipment.
[0051] The attack methods of the CASIA-MFSD dataset are divided into:
[0052] Photo attacking color-printed face photos and bending them;
[0053] The i...
Embodiment 2
[0094] refer to Figure 4 It is another embodiment of the present invention, which is different from the first embodiment in that it provides a verification test for a multi-supervised face live detection method that integrates multi-scale features, in order to add to the technical effects used in this method. The verification shows that this embodiment adopts the traditional technical solution and the method of the present invention to carry out a comparative test, and compares the test results by means of scientific demonstration, so as to verify the real effect of the method.
[0095] The experiment uses the Adam optimizer, the initial learning rate is set to 1E-4, the batch size is set to 8, the programming environment is PyTorch, and the hardware device is an NVIDIA RTX 2080Ti graphics card. In order to verify the effectiveness of the proposed multi-scale feature fusion module and multiple supervision strategy, three sets of ablation experiments are performed on the OULU-...
PUM
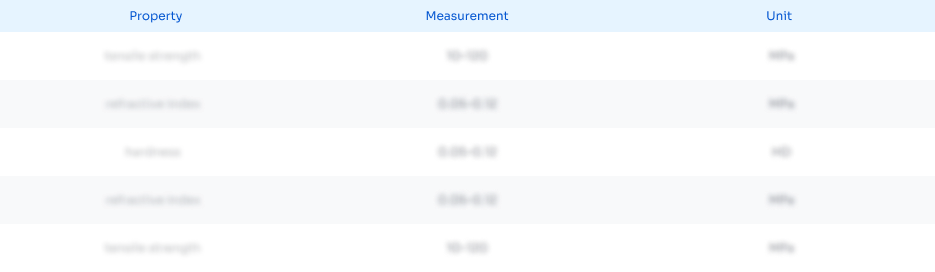
Abstract
Description
Claims
Application Information
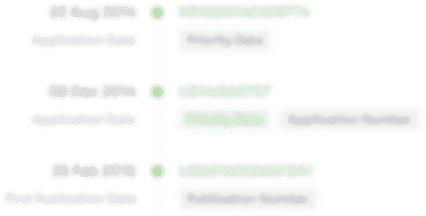
- R&D Engineer
- R&D Manager
- IP Professional
- Industry Leading Data Capabilities
- Powerful AI technology
- Patent DNA Extraction
Browse by: Latest US Patents, China's latest patents, Technical Efficacy Thesaurus, Application Domain, Technology Topic, Popular Technical Reports.
© 2024 PatSnap. All rights reserved.Legal|Privacy policy|Modern Slavery Act Transparency Statement|Sitemap|About US| Contact US: help@patsnap.com