Missing mark filling method based on sliding window sparse convolution denoising auto-encoder
A self-encoder and filling method technology, applied in the field of computer and bioinformatics, can solve the problems of inability to effectively use close-range markers, close chain relationship, over-fitting, dimension disaster, etc., to achieve improved filling accuracy, high filling accuracy, The effect of high training efficiency
- Summary
- Abstract
- Description
- Claims
- Application Information
AI Technical Summary
Problems solved by technology
Method used
Image
Examples
Embodiment Construction
[0078] The technical solutions provided by the present invention will be described in detail below with reference to specific embodiments. It should be understood that the following specific embodiments are only used to illustrate the present invention and not to limit the scope of the present invention. Additionally, the steps shown in the flowcharts of the figures may be performed in a computer system, such as a set of computer-executable instructions, and, although shown in a logical order in the flowcharts, in some cases, may be executed differently The steps shown or described are performed in the order shown herein.
[0079] The present invention provides a missing marker filling method based on sliding window sparse convolution denoising autoencoder. The overall process is as follows: figure 1 shown, including the following steps:
[0080] Step 1: First, select the first 10,000 loci of the first chromosome of rice and maize respectively, and preprocess the selected gen...
PUM
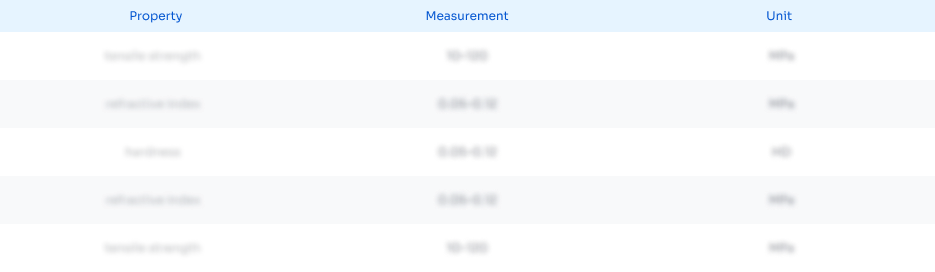
Abstract
Description
Claims
Application Information
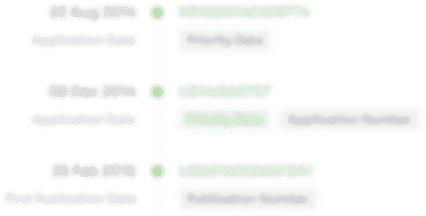
- R&D Engineer
- R&D Manager
- IP Professional
- Industry Leading Data Capabilities
- Powerful AI technology
- Patent DNA Extraction
Browse by: Latest US Patents, China's latest patents, Technical Efficacy Thesaurus, Application Domain, Technology Topic.
© 2024 PatSnap. All rights reserved.Legal|Privacy policy|Modern Slavery Act Transparency Statement|Sitemap