Flexible hybrid defect classification for semiconductor manufacturing
a hybrid defect and defect classification technology, applied in semiconductor/solid-state device testing/measurement, image enhancement, instruments, etc., can solve the problems of inflexible use of rules and defect characteristics, many deterministic methods that do not include all the characteristics of defects relevant to good classification, and fixed boundaries that often do not work well, etc., to achieve stable classification over more specimens and achieve the effect of more rapid execution
- Summary
- Abstract
- Description
- Claims
- Application Information
AI Technical Summary
Benefits of technology
Problems solved by technology
Method used
Image
Examples
Embodiment Construction
[0026] As used herein, the term “defects” refers to any anomalies that may be found on a semiconductor specimen. As used herein, the term “semiconductor specimen” is used to refer to a wafer or any other specimen known in the art such as a reticle or photomask. Although embodiments are described herein with respect to a wafer, it is to be understood that the embodiments may be used to classify defects detected on any other specimen known in the art of semiconductor manufacturing.
[0027] As used herein, the term “wafer” generally refers to substrates formed of a semiconductor or non-semiconductor material. Examples of such a semiconductor or non-semiconductor material include, but are not limited to, monocrystalline silicon, gallium arsenide, and indium phosphide. Such substrates may be commonly found and / or processed in semiconductor fabrication facilities.
[0028] A wafer may include only the substrate such as an upatterned virgin wafer. Alternatively, a wafer may include one or mor...
PUM
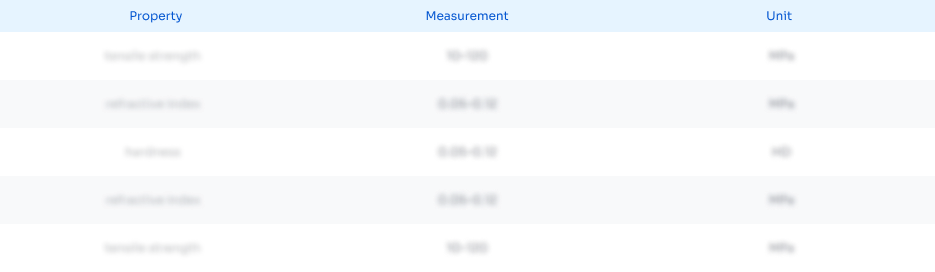
Abstract
Description
Claims
Application Information
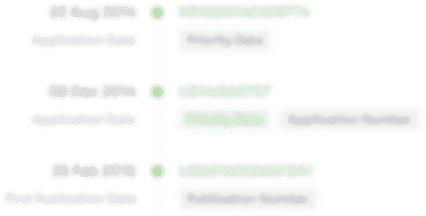
- R&D Engineer
- R&D Manager
- IP Professional
- Industry Leading Data Capabilities
- Powerful AI technology
- Patent DNA Extraction
Browse by: Latest US Patents, China's latest patents, Technical Efficacy Thesaurus, Application Domain, Technology Topic, Popular Technical Reports.
© 2024 PatSnap. All rights reserved.Legal|Privacy policy|Modern Slavery Act Transparency Statement|Sitemap|About US| Contact US: help@patsnap.com