Improved convolutional neural network-based plant leaf segmentation method
A convolutional neural network and plant leaf technology, applied in the field of image segmentation, can solve problems such as poor results, difficulty in extracting foreground objects, and mis-segmentation, so as to avoid under-segmentation and over-segmentation, overcome uneven illumination, and model parameters Small amount of effect
- Summary
- Abstract
- Description
- Claims
- Application Information
AI Technical Summary
Problems solved by technology
Method used
Image
Examples
Embodiment Construction
[0032] The present invention will be further described below in conjunction with the accompanying drawings and specific embodiments.
[0033] A kind of plant leaf segmentation method based on the improved fully convolutional neural network proposed by the present invention comprises the following steps:
[0034] (1) Test data acquisition and processing
[0035] (2) Build a fully convolutional neural network
[0036] (3) Training fully convolutional neural network
[0037] (4) Use the trained fully convolutional neural network model to achieve leaf segmentation
[0038] Described process (1) specifically comprises the following steps:
[0039] When selecting test samples, consider the complexity and diversity of the samples, such as inconsistencies in the size of the pictures in the picture collection, uneven lighting intensity, black shadows in the pictures, etc., and select diseased leaves with some diseased spots that are similar to the background color.
[0040] A total...
PUM
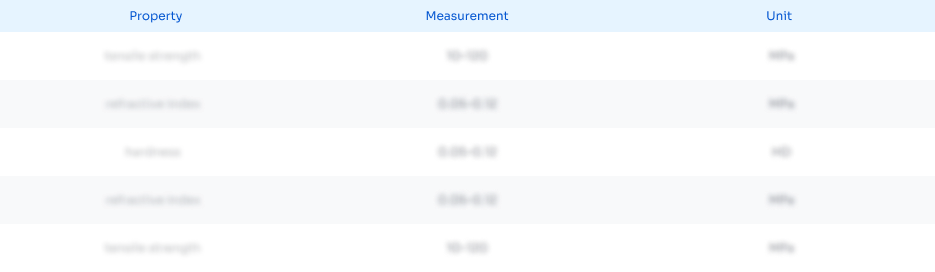
Abstract
Description
Claims
Application Information
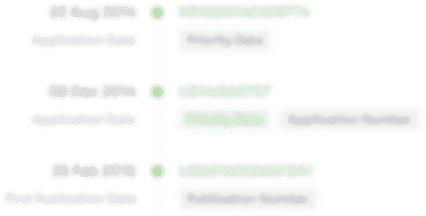
- R&D Engineer
- R&D Manager
- IP Professional
- Industry Leading Data Capabilities
- Powerful AI technology
- Patent DNA Extraction
Browse by: Latest US Patents, China's latest patents, Technical Efficacy Thesaurus, Application Domain, Technology Topic, Popular Technical Reports.
© 2024 PatSnap. All rights reserved.Legal|Privacy policy|Modern Slavery Act Transparency Statement|Sitemap|About US| Contact US: help@patsnap.com